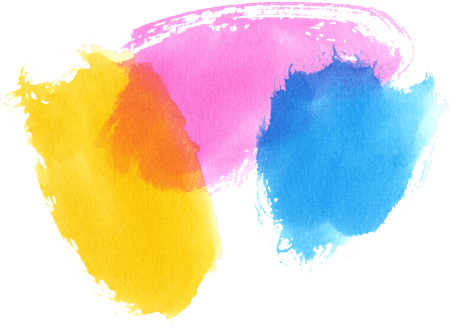
The adoption of any digital health technology should always be grounded in science, evidence-based, and subject to independent scrutiny. It’s how we can ensure medical innovation is safe and meaningfully serves clinicians and their patients.
So, we welcome the UK’s National Institute for Health and Care Excellence (NICE) guidance on our class of technology: AI software for lung nodule management on CT scans. In this article, we reflect on the conclusions of this guidance and what we can do to address some of its points.
The evidence gap
The NICE committee notes that it has found insufficient evidence to recommend the widespread adoption of AI. This conclusion is unsurprising given the technology has only been available for a few years. Also, most research efforts to date have focused on device accuracy and performance, often in order to meet the clinical validation requirements for regulatory clearance. My colleague Maurits agrees and, in his recent article, outlines our roadmap at Aidence toward generating evidence beyond analytical validity.
The guidance highlights the need for evidence showing how the use of AI solutions has a beneficial impact on clinical decision-making in the lung cancer pathway, with point 1.3 directed at “how using CAD software alongside clinician review of CT scan images affects the accuracy of detecting and measuring lung nodules.”
Our current INPACT study, funded by the NHS through the AI in Health and Care Award, will be pivotal in addressing this evidence gap. INPACT is one of the first pieces of evaluative research into the human-machine relationship in real-world clinical practice. When we conclude it, we hope to show how assistive AI technology, such as Veye Lung Nodules, can level up the quality of radiologists’ decisions to ensure all patients undergoing chest CT imaging get the best possible advice and follow-up regarding any potential lung cancer risk.
It is worth noting that the NICE guidance does not advise against AI adoption.
Cost-effectiveness
Health economics is another area where the NICE review finds limited scientific evidence supporting AI adoption. With INPACT, we will also fill in some of the blanks by helping to understand the potential gain of AI in health outcomes and associated costs.
If our AI lung nodule solution has a beneficial impact on a radiologist’s decision, we can follow this decision through the rest of the clinical pathway and into the patient outcome. However, following the evolution of a patient over a long period of time is prohibitively expensive. We will, therefore, rely on modelling, through a budget impact assessment, how Veye Lung Nodules will affect NHS finances, balancing the costs of running the software at a typical hospital against the incremental savings arising from pathway improvements.
We expect savings to result from the earlier discharge of likely benign findings and faster, more accurate reading and reporting of chest CT scans containing pulmonary nodules.
Patient anxiety
The NICE draft further states that “outside of targeted lung cancer screening, using [such] software could lead to more people being wrongly diagnosed with lung nodules, and having unnecessary CT surveillance.” It advises that this may lead to unnecessary anxiety for affected patients.
AI systems for lung nodule detection and measurements do, sometimes, identify false positives. The assumption we deduce from this guidance is that clinicians will incorrectly report on these findings. Yet we have learned from case studies and testimonials that most radiologists are prompt to dismiss false positives as they are often obvious, e.g. calcified nodules or intrapulmonary lymph nodes.
Additionally, aided by AI, the radiologist’s detection accuracy is likely to increase, enabling faster, more confident dismissal of benign nodules that may have otherwise been considered for surveillance. In this sense, automated volumetry for nodule growth assessment is a feature many radiologists appreciate in Veye. In the words of Dr Katie Johnson at Salisbury NHS, our AI solution helps her reach a “much more robust nodule follow-up in half the time.”
Furthermore, in a qualitative study we are working on as part of INPACT, many patients who were interviewed felt that the chance of an early, possibly life-saving lung cancer diagnosis outweighs the anxiety of a benign finding. This is, of course, an area for further investigation.
Thus, we question whether the risks mentioned in the guidance would manifest in clinical practice.
Ultimately, the more we can refine and focus on the cases likely to develop into lung cancer, the better for the patient. On this note, we have partnered with Google Health to deliver a medical device for malignancy scoring. Our aim is to support the early and accurate diagnosis of lung cancer and the reduction of unnecessary procedures.
A clear need
The assessment will surely constitute landmark guidance on AI for lung nodule analysis on CT imaging. We are grateful that NICE has initiated this review and given us the chance to contribute. We also hope the industry will join us in sharing their perspectives and elevating the discussion.
The draft also serves as an additional reminder of a serious pain point: the burden of lung cancer. The disease remains the deadliest cancer in the UK, largely because symptoms appear late, delaying the diagnosis until stages 3 and 4.
For the NHS to fulfil its ambition to diagnose 75% of all cancers at stages 1 or 2 by 2028, technologies such as AI for lung nodule management are indispensable.