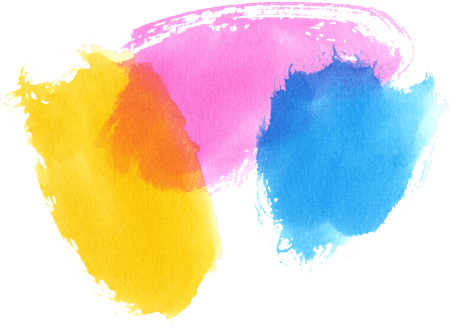
A longtime user of our AI solution, Veye Lung Nodules, once said he “feels naked without it”. This indicates its clinical utility – bringing reassurance, confidence, or comfort to the radiologist – and we were happy to hear it! But much work must be done to translate such statements into peer-reviewed evidence of Veye’s benefits in clinical practice.
At Aidence, we are building an “evidence dossier” for our AI medical devices. In a previous article, my colleague Maurits explained the framework we use to do so, outlining our existing studies and the work in progress. One of the latter is INPACT, a joint evaluation programme looking into the effect of AI on radiology decision-making in lung cancer care.
INPACT is made possible with funding from the UK’s National Health Service (NHS) through the AI in Health and Care Award. Should it demonstrate a positive impact on healthcare outcomes and cost-effectiveness, it will support the national adoption of our solution. It may improve trust and encourage the implementation of proven tools that can make a lasting difference in people’s lives.
Setting up INPACT has been a unique, complex undertaking and an excellent opportunity for us as a vendor of emerging technology to learn and derive best practices, particularly within the NHS context. In this article, we’re sharing our top tips, hoping to support further research into AI in radiology towards the benefit of physicians and patients.
INPACT basics
The INPACT study is performed in the radiology departments of six hospitals in the UK. Radiologists first analyse chest CTs unaided by Veye Lung Nodules, then provide a consecutive reading with access to Veye’s results. A radiology expert at each centre independently evaluates all cases where one or more nodules were identified by either the radiologist or Veye and compares the radiologists’ performance and confidence level. The sample consists of up to 750 unique chest CT scans per hospital, adding up to approximately 4,500 cases over six months.
We’re working on this study with the University of Edinburgh and clinical consultancy Hardian Health. The university is leading the technology-specific evaluation team (TSET), an independent academic body appointed by NHS England to oversee the programme and provide objective reporting of results. Hardian Health backs up the research for the health economics workstreams and also works closely with the six sites.
We’ve had strong support from the AI Award team and the academic TSET, which has been invaluable in helping to navigate challenges along the way.
So, what have we learned?
Our top tips

1. However long you think the project will take, double it, and then double it again!
Study design, research governance, registration and approvals, technology installation, ethics applications, participant recruitment, training, and study setup all have significant lead times. Given their interdependencies, they will have knock-on effects on the calendar.
So, build in realistic timelines from the start and validate these with the experts before committing to a “final end date”.
2. Get the right advice early on.
Real-world research, by definition, means testing a research hypothesis in a live clinical setting, where it will likely have a direct impact on the patient. Thus, it can be fraught with complications, such as blurring the distinction between research and service evaluation.
Framing the research questions and study design are non-trivial tasks. There are plenty of sources of guidance that you could, and should draw from. Here is a list of the UK advisory services:
- The Health Research Authority
- The NHS AI Lab
- The AHSN Network
- The NIHR Clinical Research Network
- The NHS Innovation Service
- The multi-agency advisory service (MAAS) for AI and data-driven technologies
In our case, talking to AI specialists at the UK Health Research Agency helped us frame the problem and finalise our study design.
3. Keep an open mind on research questions and design.
In medical imaging research, reader studies are often the go-to design for testing a particular research question. Nonetheless, they remain artificial constructs. Consequently, their utility in real-world research is perhaps limited. So, you may want to take a more creative approach to designing a study protocol.
The critical question is: how can we balance the capture of data in a real-world clinical context with minimising the burden on the already stretched clinical workforce? A randomised controlled trial may be the gold standard for measuring outcomes and impact. Yet, it is not always desirable, practical, or affordable.
There is no one-size-fits-all approach. It’s best to to play around with ideas and assess the strengths and weaknesses of different designs. Many advisory bodies (see point #2) can also provide valuable insights.
4. Map out all the parties that need to be engaged.
Moving from identifying an evidence gap to concluding and publishing a research study is a long journey, measured in years, not months. Many stakeholders will get involved along the way. This includes a mix of regulation, advisory, support and execution, with authority to allow or block a project from moving to the next stage.
Even within a single institution, like an NHS hospital, there will be multiple people to engage – research coordination, technology teams, finance, information governance, clinical and procurement, to name but a few. Much as we’d like a single point of contact in cases like this, that’s not the reality, so wide-ranging engagement is absolutely essential.
Start your project by sketching a mind map of everyone that needs to be involved. And then ask them all who else needs to participate, and how. Repeat this exercise until the mind map stops growing.
5. Involve frontline clinicians from the start.
We have been fortunate to have a good plan for our real-world research agreed upon early on with our TSET and the NHS AI Award team. But, in the build-up to starting our study and its execution, we are constantly learning new things. These are minor tweaks and improvements to, for instance, our inclusion criteria or choice of data categories.
The majority of these insights come from the clinical teams involved in the study. Although they represent issues the study team might have been able to anticipate, the clinical end users of our technology are much better placed to challenge us and spot such things up front.
Thus, if you want to collect real-world clinical evidence, make sure you have real-world clinicians advising your study team. In our example, a dedicated clinical advisor works with our clinical investigators, but many other models could work equally well.
6. Keep it simple and focus on your primary research goals.
As our study moved from design to execution, it was not uncommon to ask ourselves questions like “[x] thing is interesting, maybe we should explore [y]?” or “Would [a] be able to tell us anything more about [b] if we do [c]?”. Be warned – that way leads to scope creep! It requires discipline to stay focused on the primary aims of the research, and, fortunately, our TSET has maintained a laser focus on these aims.
Before commencing a real-world study, do one last sense check of the primary research questions and the study’s aims. Are these truly the questions you want answers to? If so, stick to them, but don’t discard other ideas you might have as you progress. Collect them somewhere for consideration in future research.
7. Understand the decision authority to proceed.
The hardest part of our research journey (so far, at least) has been getting started. We had sketched out some initial timelines which we thought were realistic (see point #1). Still, we quickly realised that getting the green light to proceed involved navigating several stakeholders (point #4), which all took time.
A further complication was that our project was sponsored by a Scottish body, with different governance arrangements from the English partner sites. At times, even our TSET (with its vast institutional experience of running clinical research) was unclear on who had authority over which decision. It doesn’t help that many of the decisions required to initiate clinical research are interdependent. A won’t sign B until C has signed D, which depends on A approving E.
The takeaway: document carefully, in the form of a decision tree, who has decision authority over your project, and how that authority is exercised. Note the lead time, prerequisites, and any interdependencies. Doing this upfront will take time, but your project will run much more smoothly in the long term.
8. Incentivise the research effort.
To run a real-world clinical study, you need real-world clinical people. However, NHS clinicians are under immense pressure from multiple systemic challenges. And, in almost every aspect of care, they go above and beyond.
Therefore, one of our early principles was not to take their participation in our research for granted. We recognise the time and commitment required to support a study like ours. In some cases, this might simply be a recognition of their efforts in any resultant research publications. But principally, we want to ensure the financial incentive is appropriate—institutionally and personally—for the extra effort required to support the research.
If your research involves the use of clinician time (which it invariably will), discuss with them what a fair system of remuneration and recognition might look like and build into your study process.
9. Think about the meaning of “real world.”
This might sound abstract, but it is, nonetheless, key to understanding the value of any research. You could have the best and most performant AI algorithms in the world and prove, without question, that clinicians using them will be consistently more accurate or work faster. But that doesn’t answer the pivotal question: “So what?”
What does that mean in practical terms? How many patients will benefit, and how? What does it do for costs and efficiency? Is the solution easy to use in practice, and will it actually be used?
That is why it is so important to think of clinical utility and cost-effectiveness in the context of real-world clinical care. We discarded many of our early research ideas because they told us nothing about what would happen in real life.
You may not be able to reliably test a solution in every real-world dimension. So, be clear about which dimensions are important for your evidence gaps and design around those. These may be efficiency, clinical decision-making, ease of use, potential bias, etc.
10. Don’t plan to do everything in sequential steps.
Our final advice is one of practicality more than anything else. As an AI Award-funded programme, we are expected to wrap up our project in line with the funding award agreement. However, as timelines have drifted to the right (point #1 again), there is less and less time at the end of the project for data collation, results analysis, interpretation, and reporting.
Our solution is to bring forward as much work as possible from these final stages of the project to minimise the time required for the last steps. That means prepping our analytics work with dummy data, pre-writing elements of our research papers that are not dependent on the actual results, and generally ensuring that we can still hit our deadline for deliverables without compromising the study’s integrity.
It’s an old cliche, but there is merit in “starting with the end in mind”. If that means drafting a mock-up scientific paper before you even start your study, that’s likely to be a good course of action. It will expose any weaknesses in your methodology or data strategy and ensure everyone is sighted on what you need to achieve your aims. Don’t wait until the end of the project to start thinking about what it all means.
Never forget the patient!
This is our “bonus” eleventh tip.
When conducting scientific research, it is easy to get lost in theory, governance, and statistics. So it behoves researchers to continually focus on those who matter most: the patients and citizens at the receiving end of all medical technology innovations.
It is best practice to involve patient and public representation throughout a research programme, from conception and study design to execution and analysis. In the NHS, there are plenty of resources to help create effective patient-focused research. We recommend the NIHR Learning for Involvement website as a great place to start.
Active engagement with patients and the public helps us never lose sight of our driving mission: to give all lung cancer patients a fighting chance.
Thanks are due
All the learnings we derived from setting up a research programme into AI within the NHS will help us to grow as a company and streamline our following projects. They may also allow other technologies facing a similar conundrum to, for instance, understand how and where to get started.
Preliminary results of INPACT will be made available to the NHS AI Award team in March 2023, and we will publish our findings thereafter. We want to thank all the organisations involved, especially the radiologists in the study sites. They are helping us build the body of evidence that will realise patient benefits across the whole NHS.