Development & Validation
The clinical performance of Veye Lung Nodules in nodule detection, classification, quantification, and growth rate estimation has been validated in a study performed by the University of Edinburgh and NHS Lothian.
(see Clinical Validation Study segment)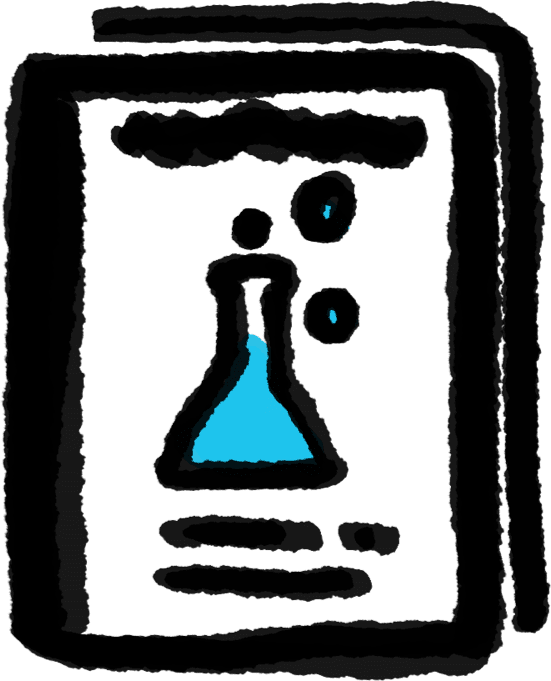
Veye Lung Nodules has an overall accuracy of 95.96% and a kappa score of 0.81 for determining the composition (solid, sub-solid) of a pulmonary nodule.
Readings aided by Veye Lung Nodules yielded a 10% higher sensitivity, compared to unaided readings, while hardly affecting the false positive rate.
The average discrepancy between Veye Lung Nodules and a radiologist (1.24) is lower than the average discrepancy among radiologists themselves (1.30) in volume growth assessment.
Research
The clinical validation study of Veye Lung Nodules1 was conducted at the Royal Infirmary of Edinburgh hospital (NHS Lothian) and the University of Edinburgh under the leadership of Dr. Prof. John Murchison.
Validation of a deep learning computer aided system for CT based lung nodule detection, classification and quantification and growth rate estimation in a routine clinical population
John T. Murchison2, Gillian Ritchie2, David Senyszak3, Edwin J.R. van Beek2, 3
1Veye Lung Nodules was known as Veye Chest at the time of the study.
2Department of Radiology, Royal Infirmary of Edinburgh, Edinburgh, UK.
3Edinburgh Imaging facility QMRI, University of Edinburgh, Edinburgh, UK.
Posters
The results of the clinical validation study were showcased during the 2019 European Congress of Radiology (ECR) as two e-posters and two abstract presentations.
Evaluation of deep learning software tool for CT based lung nodule growth assessment
Evaluation of deep learning software tool for CT based lung nodule segmentation
AI modelling basics
Medical imaging AI “learns” from radiologists. The datasets that are the foundation of an AI clinical application consist of scans manually annotated by radiologists (e.g. by outlining a lung nodule or providing its classification). There are three annotated sets of medical images required to develop an AI solution:
Training: The AI model directly learns from the data in the training dataset. A good training dataset contains a large number of scans from varied sources.
Validation: A subset of the training dataset is used to assess the performance of all the trained models on a real-world population and to pick the best model to check against the test set.
Testing: A new dataset that is not part of the model’s learning is used to provide an independent evaluation of the clinical performance of the AI model.
The datasets
Veye Lung Nodules’ detection feature was trained with 45,000 chest CT scans provided by the National Institute of Health (NIH) from the National Lung Screening Trial (NLST) in the United States. This dataset was enhanced with manual segmentations of each nodule by certified Dutch radiologists.
The clinical performance of Veye Lung Nodules has been validated using two databases:
The LIDC/IDRI, a database merged from the Lung Image dataset Consortium (LIDC) and the Image Database Resource Initiative (IDRI). The database was designed to support the development of Computer-Aided Diagnosis (CAD) software and consisted of over 1,000 scans and annotations by several experienced radiologists.
A database was developed in cooperation with the University of Edinburgh during a clinical trial. The data came from the UK’s National Health Service (NHS) Lothian database and included over 333 chest CT scans. The reference standard was set by two expert thoracic radiologists.
Detection
The Free-Response Receiver Operating Curve (FROC) represents the detection performance for nodules over 3mm and 5mm in sensitivity levels (Y-axis) and corresponding false positive rates (X-axis).
The FROC based on a dataset with 1,000 CT scans, 1,300 nodules that were read by 4 thoracic radiologists.
Volume Growth Assessment
Multiple studies3 indicate that nodule volumetric analysis, including volume growth and doubling time, might offer better insight into the growth or decline of a lesion compared to measurements based on diameters.
For our claimed accuracy, we compared the discrepancies in the average volume growth in two cases: with the results of Veye Lung Nodules and the radiologists’ annotations; and amongst the annotations of three radiologists.
Data on file shows that the average discrepancy between Veye Lung Nodules and the radiologist was lower than the average discrepancy among the radiologists themselves (1.24 to 1.30).
3For example Mozley, P D et al. “Change in lung tumor volume as a biomarker of treatment re- sponse: a critical review of the evidence.” Annals of oncology: official journal of the European Society for Medical Oncology vol. 21,9 (2010):1751-1755. doi:10.1093/annonc/mdq051 and Hayes SA, Pietanza MC, O'Driscoll D, et al. “Comparison of CT volumetric measurement with RECIST response in patients with lung cancer.” Eur J Radiol. 2016;85(3):524-533. doi:10.1016/j.ejrad.2015.12.019.