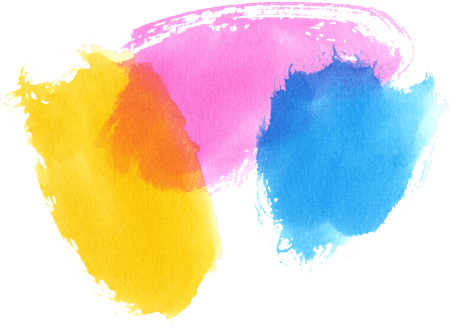
Project INPACT is go!, reads a recent tweet from clinical consultancy Hardian Health. We have officially launched novel research into a fascinating yet understudied area: the human-machine relationship. Project INPACT (Investigating Nodule Protocol Adherence using CADe/x Technology) will give us insights into the impact of an artificial intelligence (AI) medical solution on physicians’ decision-making.
Most scientific literature on AI clinical applications focuses on their performance, for instance showing a device is safe, effective, and works as intended. INPACT, on the other hand, is one of the few studies examining AI in actual clinical practice: the reporting of chest CT scans in radiology departments across UK hospitals. The project is a collaboration between Aidence, Hardian Health, and the University of Edinburgh, with funding from the UK’s National Health Service (NHS) through the AI in Health and Care Award.
In this article, we explain the reasoning behind and the design of INPACT.
AI for early lung cancer detection
Time is crucial in lung cancer. Symptoms develop late, delaying the diagnosis until the disease has already advanced. Consequently, lung cancer is associated with the poorest survival rate among cancers worldwide.
Early detection is the best chance of improving outcomes for lung cancer patients. There are two main opportunities to ensure it: targeted screening programmes inviting individuals at-risk for a low-dose chest scan, and the reporting and follow-up of indeterminate lung nodules found incidentally in clinical practice.
The INPACT study will focus on the latter. In routine practice, patients undergo scans for varied medical indications. If a radiologist finds an abnormality in the lungs – a nodule possibly indicative of early lung cancer – they make an assessment of the nodule’s characteristics and give a recommendation for managing its evolution, e.g. follow-up with a CT scan in three months.
Most lung nodules detected in this way are benign, but some are cancerous. Their identification and follow-up are essential to an early lung cancer diagnosis, when a cure may still be possible. However, these tasks are challenging for physicians, requiring them to spot millimetric lesions with the naked eye, characterise, and measure or segment them (semi-)manually. For a workforce already under strain, it is additional, demanding work. Moreover, since radiologists are not primarily looking for lung nodules on routine scans, they may miss possibly threatening abnormalities.
AI tools have the potential to identify incidental pulmonary nodules on chest CTs, classify them, and provide accurate measurements to assess growth. As part of the INPACT project, radiologists will report on these findings using AI-based solution Veye Lung Nodule, our CE certified second or concurrent reader, fully integrated into the hospital IT infrastructure. The aim is to investigate this solution’s effect on physicians’ follow-up decisions.
The reasoning behind INPACT
We can say it best with a basic Venn diagram showcasing the position of AI medical solutions in healthcare. On the left, clinical validation research is the topic of many publications aiming to demonstrate the performance of an application. For commercially available medical devices, it is also a regulatory requirement. (You can find out more about the validation study which confirmed Veye Lung Nodules’ performance on this page.)
On the right, clinical outcomes represent the endpoints we are trying to influence by introducing AI devices in care pathways. This area is largely unexplored because AI technology is still in its early stages. We have not had the time or resources to complete extensive clinical trials, often spanning over many years, to assess if patients whose doctors use AI systems receive a more precise or timely diagnosis or care.
With the INPACT project, we zoom in on the middle: the real-world use of AI in clinical practice.

Our assumption is that radiologists using an AI-based solution for lung nodule management and reporting may come to a different follow-up recommendation than they would have without AI.
Furthermore, this different follow-up recommendation would be more in line with what an experienced radiologist specialising in chest imaging would have given. It is even possible that, in the absence of the supporting tool, radiologists would have missed small nodules or come up with inaccurate diameter or volume measurements.
Participating hospitals
The INPACT study will be performed in the radiology departments of six hospitals in the UK: NHS Lothian / Royal Infirmary of Edinburgh, University Hospital Southampton NHS Foundation Trust (FT), Royal Cornwall Hospitals NHS Trust, North West Anglia NHS FT, Liverpool Heart and Chest Hospital NHS FT, Royal United Hospitals Bath NHS FT.

Having closely worked with radiologists to develop and improve our solutions, we understand how busy they are, and we have no intention of increasing their workload. So, a primary consideration in the design of this study has been ensuring that radiologists spend the least amount of time possible on the study while collecting enough data to make it feasible.
Dr Rishi Ramaesh, consultant radiologist at NHS Lothian and principal investigator, explained his motivation for participating in the study:
“The INPACT study is a highly novel and exciting evaluation that will demonstrate tangible and real world benefits of using artificial intelligence in clinical radiology practice. The study aims to show that radiologists using AI in their clinical practice are safer and more efficient, along with clear benefits to patients and healthcare systems.
The results of the INPACT study are sure to strengthen the case for widespread adoption of AI tools into the NHS.”
The study design
The sample of cases for this study will consist of up to 750 unique chest CT scans per centre, adding up to approximately 4,500 cases in total over six months. INPACT will be a prospective and comparative study, with the following design:
Aided and unaided readings
The radiologists at the participating centres will assess the eligible CT scans for lung nodules and provide their recommendations according to the British Thoracic Society (BTS) guidelines, as they would normally in routine clinical practice.
First, they will analyse the image unaided by Veye Lung Nodules and decide on the next steps. Then, they will provide a consecutive reading with access to Veye’s results: detected nodules, their type (solid or sub-solid), size (diameter and volume) and growth (growth percentage and volume doubling time from the prior scan, if available). Here is an example of a report generated by Veye Lung Nodules:

After the aided reading, the radiologist may adjust their initial follow-up or management recommendation and provide an explanation for the change and feedback on Veye’s results. To streamline this step, we created a simple case report form which should only take a couple of minutes to fill in.
A radiology expert at each centre – i.e. a consultant with around ten years of experience in thoracic imaging – will independently evaluate all cases where one or more nodules were identified by either the radiologist or Veye. This expert may also consult with a pulmonologist on complicated cases. With readings aided by Veye Lung Nodules, it is likely to reach a higher agreement between the expert and the less specialised radiologists reading the scans.
We hypothesise that the AI-based automated detection, classification and quantification of lung nodules will reduce the influence of human measurement errors and allow radiologists to make a better recommendation for managing the nodules.
A mixed-method approach
Once collected, we will look at the results from two different perspectives:
- Quantitative
The radiologist’s potential performance boost when using Veye Lung Nodules. We will gather this data by analysing the filled-in forms.
- Qualitative
The level of confidence that the radiologist has in understanding and accepting the outputs of Veye and using these outputs in their clinical decision-making. To obtain this data, researchers from the School of Social and Political Science at the University of Edinburgh will conduct interviews with participating radiologists before and after using Veye Lung Nodules.
Health economics
If Veye Lung Nodules has a beneficial impact on a radiologists’ decision, we can follow this decision through the rest of the clinical pathway and into the patient outcome. This would allow us to pinpoint the benefit of using the device for early detection and possibly life-saving treatment.
For this purpose, our partners at Hardian Health will perform health economics and cost-effectiveness modelling. Cost-effectiveness analyses (CEA) are recognised as the gold standard in health economic evaluations. The National Institute for Health and Care Excellence (NICE), for example, is considering it for the upcoming Evidence Standards Framework for digital health technologies.
Using the outputs of the quantitative research, the researchers will extrapolate their impact using health economics modelling techniques.
Dr Hugh Harvey, Managing Director at Hardian Health, emphasises the value of this part of the study design:
“Clinical impact is vitally important to demonstrate in order to gain trust from end-users, but health economic evaluations are equally as important in helping gain trust from payers, especially in state-funded and evidence-based healthcare systems such as the NHS.”
The below image is a summary of the INPACT approach:

A blueprint for future research
The pace at which AI medical devices are brought to market exceeds the time and budget for clinical trials assessing their impact. Since the NHS is at the forefront of adopting new technologies, it needs more agile ways of evaluating AI solutions in the real world. NICE, in fact, is also consulting on a real-world evidence framework.
INPACT might serve as an example of such a framework. The design of the study and the lessons we will learn may be generalisable into a blueprint for assessing the application of AI in radiology. Its strength is that it does not require a large infrastructure or extended period. However, it will likely yield valuable results, allowing us to understand how AI makes a difference in patient outcomes.
One first learning from the creation of INPACT is already practical: the project demonstrates that it is possible to set up a real-world AI evaluation programme at a relatively low cost and within a tight timeframe.
Stay tuned
The results of INPACT are planned for the end of 2022. Our expectations are high, as is our excitement. We believe that the results will be a turning point toward medical imaging AI becoming part of the standard of care.
At the same time, we are taking part in different investigations into and conversations around the use of AI in healthcare. We recently contributed to a report from Health Education England, which did field research on clinicians’ confidence in AI medical devices. Furthermore, our Medical Director, Lizzie Barclay, has shared her expertise with the Ada Lovelace Institute on their proposal for an algorithmic impact assessment framework for data access within the NHS.
For regular updates on our progress, subscribe to our newsletter or follow us on LinkedIn or Twitter.