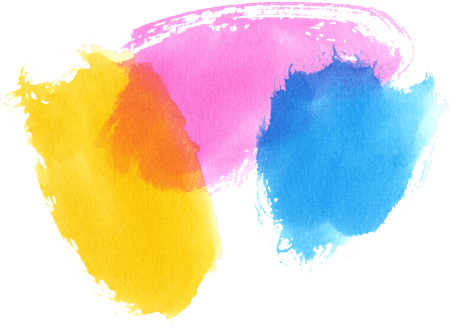
When it comes to medical imaging AI, the proof is in the clinical studies. Or should be. While a quick PubMed search of “AI” and “radiology” returns more than 10,000 hits, only 36 of the 100 CE-marked AI solutions assessed by Kicky Van Leeuwen et al. are supported by peer-reviewed scientific publications.
Most of these studies focus on mere model performance, which is often insufficient to distil how the solution benefits clinical practice. Research into the impact of AI on physician decision-making, patient outcomes, or cost-effectiveness is just as relevant to determine its value.
This article outlines our approach to building up a body of clinical evidence for the validity and utility of Veye Lung Nodules, our AI solution for pulmonary nodule management on chest CTs. By sharing our example, we also hope to provide radiologists looking to adopt AI with a practical guide for assessing available publications.
The evidence development roadmap
With radiology AI still in its early stages, there is no definite track of requirements to prove its worth. Thus, we took inspiration from existing examples, such as the evidence development frameworks for biomedical diagnostic tests and digital health technologies, to create our own pathway.
The roadmap to building evidence for Veye Lung Nodules has four stops leading to adoption, as shown below. Good to note that some of these studies are also required to access the healthcare market via certification, such as the EU’s Medical Device Regulation.

Let’s zoom in on what each of these steps entails. The next section will show examples for each category.
1. Analytical validity: The stand-alone model performance
This foundational stage involves showing the model’s stand-alone performance: how well it does the task for which it was trained, e.g., detecting lung nodules on chest CTs.
Analytical validity studies serve three goals:
- Report on the model’s development;
- Assess its performance on “unseen” data (external validation);
- Address its generalisability to different scanners and patient populations.
The STARD guidelines, supplemented by the TRIPOD statement, can guide researchers in the appropriate reporting of prediction models or diagnostic tests. Adaptations specific to AI interventions are, nonetheless, necessary.
2. Clinical validity: The impact on the radiologist’s performance
Radiology AI today is, with very few exceptions, assistive technology. It supports radiologists in their work rather than autonomously performing any of their tasks. Thus, at this stage, we look at the human-machine interaction.
Essentially, we view the AI not just as a performing model but as a medical device providing a solution to a clinical challenge. Determining its validity often equals demonstrating that the solution enhances the radiologist’s assessment. This means, for example, that the radiologist detects more actionable pulmonary nodules when aided by AI than without it.
3. Clinical utility: The impact on the patient, radiologists, and healthcare outcomes
Medical imaging is often the starting point of a care pathway. Subsequently, AI’s improvements in the radiology workflow may have a major effect down this pathway.
For instance, our AI solution delivers the pulmonary nodules’ location, composition, and size metrics. The availability of this analysis may improve the radiologists’ performance, i.e., increase their speed and accuracy. The outcomes could be significant. Fewer unnecessary follow-up CT scans mean less patient anxiety, time savings, and lower costs. The early detection of a malignant lung nodule would be a life-changing result for the patient.
Evidence of the downstream outcomes of the solution’s implementation is as fundamental for its clinical adoption as pure regulatory body-approved market access. This stage may include varied study designs, from small retrospective formats addressing specific questions to larger prospective (randomised) trials establishing high-level evidence, if resources allow it.
Depending on the study design, research in this phase can be guided by the CONSORT, SPIRIT or STROBE guidelines.
4. Cost-effectiveness: The impact on healthcare costs
Finally, cost-effectiveness can be estimated by considering all previously obtained evidence as assumptions in economic and cost-effectiveness modelling exercises.
Following the downstream improvements mentioned above, the overall cost impact of a tool like Veye Lung Nodules can be considerable. By detecting one or two malignant nodules the radiologist alone would have missed, a hospital could save tens of thousands of euros in cancer treatment costs annually.
Studies on Veye Lung Nodules
At Aidence, we’re in the midst of our effort to build an evidence base for our AI lung nodule solution. Here is a glimpse into our research library today, in line with the framework above.
Study for CE marking
The clinical performance of Veye Lung Nodules was validated for CE marking in 2018 in a retrospective reader study in collaboration with the University of Edinburgh and NHS Lothian. The study was published in 2022 as a peer-reviewed article by Murchison et al..
The Free-Response Receiver Operating Characteristic (FROC) curve below illustrates the accuracy of Veye’s models. The curve plots the sensitivity against the mean false positive findings per scan, over various possible model thresholds and device operating points. On default settings, Veye detects nodules between 3 and 30 mm at a sensitivity of 91% at the cost of one false positive per scan.

Murchison et al. also showed that radiologists detect more lung nodules that may need follow-up when using Veye Lung Nodules. Unaided by Veye, the radiologists reported a sensitivity of 71.9% for detecting actionable nodules on 273 scans. This increased to 80.3% with Veye’s support, an almost 10% sensitivity boost.
Another notable metric is the Dice Similarity Coefficient (DSC), which describes the similarity between two segmentations: those made by radiologists and those by Veye Lung Nodules. Murchison et al. reported a mean DSC of 0.86 for Veye’s and the radiologist’s segmentations of the found lung nodules; the DSC between the radiologist’s segmentations was 0.83. So, the overlap of segmentations between Veye Lung Nodules and a radiologist seems greater than among radiologists themselves.
Moreover, the average difference in diameter measurements between radiologists and Veye was ± 1.17 mm. This was similar to the differences among radiologists (± 1.15 mm).
Stand-alone performance confirmed
Martins Jarnalo et al. reported their own external validation of Veye’s stand-alone performance in routine practice. They retrospectively analysed 145 patient CT scans from a teaching hospital in the Netherlands. Veye’s sensitivity was 88%, with a negative predictive value of 95%. 90% of the nodule diameters provided by Veye differed by one mm or less from the radiologists’ measurements.
These results indicate strong performance, in line with the CE marking specifications. The study was published in Clinical Radiology.

The effects of CT reconstruction settings on Veye Lung Nodules’ output were further addressed by Blazis et al. in a European Journal of Radiology study. While different reconstruction settings impacted Veye’s output, performance was as expected with the clinically preferred reconstruction settings for chest CTs.
Exploring the clinical utility
As mentioned, the value of an AI solution often exceeds its primary task. Veye Lung Nodules aids in detecting, classifying, quantifying, and assessing the growth of pulmonary nodules. Assisted by its clinical features, radiologists may make better decisions, which will have cascading effects.
We started exploring some of these effects together with physician-scientists at various hospitals. Here are the main projects:
Efficiency study
Hempel et al. first touched upon whether Veye Lung Nodules affects radiologists’ agreement in a small pilot study. It showed that the readers were more likely to reach a consensus on patient management recommendations when using Veye. What’s more, they came to their conclusion almost 40% faster, despite a washout period of six months. To learn more about findings, read this summary.
INPACT
These efficiency study results inspired a sizeable prospective study led by a team at the University of Edinburgh and NHS Lothian: INPACT. It aims to determine if reporting radiologists using Veye are more likely to agree with experienced thoracic radiologists when managing lung nodules in routine clinical practice. The project is live in six UK sites, with the NHS’ support through the AI award.
In collaboration with Hardian Health, we will further use the outcomes and all other evidence in a cost-effectiveness analysis.
Research into volumetry
At the 2022 European Congress of Radiology, I. Gimbel shared the preliminary results of another promising study. It indicated that the availability of Veye’s volumetric analysis could have led to earlier discharges in 18.5% of patients. The final results are pending.
On our way
Although many companies offer AI solutions for radiology, evidence for these at various stages is often lacking. One reason is that, though widely discussed, the implementation of medical imaging AI is still relatively new. It takes time to collect enough data and obtain the resources to do so in a clinical research setting, be it retrospective or prospective. Public-private collaborations are crucial, but can be time-consuming.
At Aidence, we will continue to encourage, facilitate, and collaborate in research initiatives to substantiate our clinical claims further. Clinical research is also vital in post-market surveillance, next to customer feedback and audits.
Soon, we will be able to address every step in our evidence development pathway. Undoubtedly, more questions will arise along the way, and we will be ready to tackle those as well!