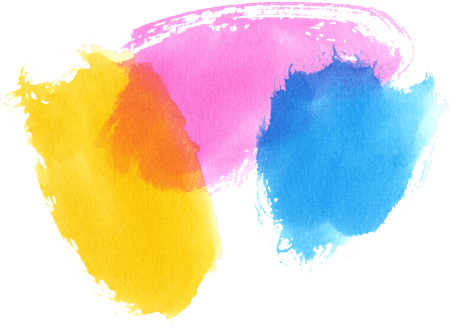
“Welcome to the era of autonomous AI in medical imaging,” read an announcement from an AI company last April. They had obtained the CE mark for a tool intended to report on normal chest X-rays – without involving a radiologist.
AI autonomy in healthcare was not an entirely new development. Last year, the World Health Organization (WHO) recommended using computers as an alternative to human interpretation of X-rays for screening and triaging of tuberculosis. In the US, an FDA-approved AI device autonomously diagnoses diabetes-related eye problems.
Still, following this milestone, our industry reopened the debate around the meaning of autonomy and the safety of an AI reporting on medical images autonomously. The medical community was particularly triggered by what constitutes a “normal” scan. Dr Lauren Oakden-Rayner, for example, wrote a notable blog on the subject.
Where do we stand when it comes to autonomous AI in medical imaging? In this article, we give some insights and explore new dilemmas. We argue that there is a strong use case for an autonomous AI ruling out scans in lung cancer screening (LCS) but that we must address some big questions before building such a system – such as defining “normal.”
What is autonomous AI?
AI autonomy means no “human in the loop“: systems that act independently of humans, e.g., self-driving cars and smart manufacturing robots. In medical imaging, it is the software that performs a clinical task, typically without involving an expert physician, i.e., a radiologist.
In the example mentioned above, if the AI predicts that an X-ray has no abnormalities, it automatically generates a report and returns it to the PACS. The radiologist does not review it. They only receive the X-rays about which the AI is uncertain. Depending on the hospital’s workflow (Radiology Information System – RIS; or Picture Archiving and Communication System- PACS), the system may send the automatically generated reports to the RIS.
The logical first question is: what is the impact on patients? The AI system will allow physicians to focus more on suspicious cases and, thus, on the patients who may need more time and help. But it will also dismiss scans without expert medical interpretation. What if some of these would have required a review?
Why do we need autonomous AI?
Since autonomous AI medical devices impact patient care, it’s worth questioning if we genuinely need them. In our view, yes, for one major reason: to make early cancer detection through targeted screening of large populations feasible. Prof Dr Bram van Ginneken from Radboud University Medical Center emphasised this point at this year’s Fleischner Society meeting.
More and more screening programmes are starting while the radiology workforce is understaffed (for example, in the UK). To deliver on their life-saving goals, we must deal with the additional scan volumes without putting more pressure on radiologists.
Most screening scans present no abnormalities yet take significant review and reporting time. A rule-out AI system could dismiss the scans with no actionable findings, reducing the radiologists’ workload and allowing them to focus on the cases where their expertise is indispensable. This is detection autonomy; the radiologist is the one making a diagnosis, i.e., confirming the presence of a disease and deciding on the follow-up.
Lung cancer screening is a particularly relevant opportunity to implement a rule-out system. Supported by positive evidence such as the NELSON and NLST trials, initiatives are increasingly being implemented in Europe. Recent data indicates that rolling out a population-based programme here would prevent 18,000 premature deaths at a cost of 937 million euros. The US has expanded eligibility criteria as the country is also scaling up screening.
As Aidence, we have a proven track record of deploying AI for lung nodule management in screening sites across the UK’s Targeted Lung Health Checks. By joining RadNet, we aim to expand our support and, down the line, build a complementary, autonomous AI for LCS.
How can we get there? As with the development of all our solutions, let’s start with a clear understanding of the clinical setting.
How do you detect “normal” on scans?
It’s perhaps the trickiest word in radiology: “normal”. In this Q&A, Dr Maskell describes it as “slippery” but attempts a definition:
“What we are describing as “normal” is probably defined better as the absence of abnormality, or at least the absence of an abnormality worth mentioning.”
Diving into the concept of detecting normality on scans, Dr Oakden-Rayner draws an analogy with detecting a safe environment around a self-driving car. Why have automobile companies not developed a safety recognition system yet? A possible reason:
“Safe conditions” are not a homogeneous class, but instead are the absence of an enormous range of visually distinct phenomena.”
The same complexity applies to medical imaging. Dr Oakden-Rayner’s article uses the example of the heterogeneous nature of bone fractures. They have different shapes and radiological features and manifest in different body locations. Also, we might add, the appearance of bone fractures with the same shape, features, and location may vary across cases or scanning protocols.
A “normal scan detector” that determines the absence of all possible bone fractures is a detector that recognises all possible bone fractures – anywhere in the body! This system would have to be tested on a dataset that captures the full spectrum of bone fractures. We’d need to assess the negative predictive value for all the diseases to exclude, i.e., the probability that dismissed scans truly don’t have any signs of these diseases. Not to mention that the system would also need to evaluate the quality of the images to identify issues that may interfere with its decision.
Thus, building a “normal scan detector” is highly complex. How can we take on this complexity in the case of CTs in LCS? By also starting from the slippery word.
What is “normal” in LCS?
Unlike routine clinical practice, where patients present for a scan with various medical complaints, LCS targets asymptomatic individuals, looking for early signs of lung cancer. So, an intuitive answer to “What is a normal scan in lung cancer screening?” is: “One that does not show signs of lung cancer”. It is, however, more complicated.
Although most screening scans will not show any signs of disease, different types of conditions can be visible on a chest CT, apart from those related to the goal of the screening programme. Many of these will not be actionable, but some may affect the patient’s health. There is currently no international consensus on reporting and following up on incidental findings in LCS.
Some guidelines, such as NHS England’s, advise radiologists to report multiple findings. Others recommend actioning only specific incidentals because following up on most of them would lead to unnecessary investigations, additional costs, and patient anxiety. The British Society of Thoracic Imaging and the Royal College of Radiology leaves the responsibility of weighing the benefits and harms to the radiologist.
Nonetheless, there is agreement on the specific threatening diseases that physicians will most commonly encounter, namely the big three: lung cancer, of course, chronic obstructive pulmonary disease (COPD), and coronary artery disease (CAD). To these, clinicians add osteoporosis, all the more relevant with research showing the opportunity to improve its detection during LCS. The likelihood of other actionable disorders in this setting is much lower.
We could, thus, argue that a normal CT scan in LCS excludes the major diseases. Yet, a consensus is needed before we can build on this definition. Ultimately, as an AI manufacturer, we rely on clear guidelines and workflows to successfully deliver solutions that are embraced by the medical community.

What is the benefit/risk trade-off?
There is a further argument for agreeing on a definition of “normal” as excluding a limited number of findings: the feasibility of the AI solution, to the benefit of patients.
An AI-based rule-out tool for normal CTs would enable large-scale LCS programmes that improve population health. For instance, 14 million people in the US are eligible for lung screening. Supporting the radiology workload with AI could improve outcomes for hundreds of thousands of people.
However, if this rule-out AI system were required to detect all the rare diseases, even those that one patient in 100,000 may have, it is not something we can deliver, neither now nor anytime soon. The rarer the abnormality to validate, the harder it is to find sufficient cases on which to train and test the algorithm. For example, if our definition of “normal” included “absence of a Playmobil traffic cone in the lungs,” we would need sufficient test scans to power a clinical study to prove that – which is not achievable when there is only one known case.
It would simply be counterproductive, if not impossible with today’s resources, to develop an AI system that excludes – thus, also detects – all incidental findings on a chest CT. But excluding the main diseases, that we can do.
It is a matter of weighing the benefits against the risks. This crucial trade-off in adopting autonomous AI for LCS leads us back to patient impact: Should we save more lives by catching more cancers and the main incidentals but accept that we’ll occasionally miss rare diseases?
How can we build an autonomous AI for lung screening?
Assuming the medical community confirms that the benefits of an AI rule-out system for the most common and life-threatening findings outweigh the risks, what’s next?
Development is no easy feat. The solution must show appropriate sensitivity and negative predictive value for all the diseases that it must rule out. We would need a lot of high-quality data to create this capability – we can’t even estimate how much. These datasets should be carefully curated to cover each abnormality and be validated on a large and diverse patient population.
The second challenge: designing a clinical study. When would this algorithm be accurate enough? We think the most cautious way to validate autonomous AI for chest CTs is by first creating a triage system to prioritise suspicious scans in the radiology worklist. This would follow the same logic: flag the more urgent scans and label the others as normal. However, the triage system would solely present the scans in a different order rather than dismiss the normal ones. The radiologist would still review all the CTs, starting from the top of the list.
This step would allow us to use the real-world clinical setting to study the performance of the future autonomous AI solution. The triaging tool would give us the opportunity to validate the output of the AI, namely the radiologists’ agreement with the differentiation between normal and abnormal.
What about laws and regulations?
At Aidence, we know an AI medical device is much more than a robust algorithm. Since there is a patient behind everything we do, quality assurance and regulatory compliance are essential to providing a safe and effective device.
The level of autonomy is central to the regulatory assessment of the potential clinical risks of using AI. The EU Medical Device Regulation (MDR) classifies AI software as classes I, IIa, IIb, or III based on the perceived risk of patient harm posed by the information it returns and how physicians use it. (More on classification under the MDR in my colleague Leon’s article.)
Autonomous AI falls under the highest class because it provides a clinical decision without a clinician’s interpretation. Should its assessment be incorrect, the impact on the patient might be significant. The AI act, furthermore, requires “human oversight” for all high-risk AI systems.
This further raises questions about liability insurance and the ability to sue an AI. Our take is that the healthcare provider is the deciding factor. If a hospital adopts an AI to fulfil a physician’s task, they assume the liability for the patients’ care, just like they would when hiring a doctor. If the device malfunctions, the manufacturer is responsible for its impact, as is the case today.
The legal and regulatory complexities made Dr Hugh Harvey at Hardian Health conclude that, although autonomous AI is now available, we’ve yet to see how fast the relevant laws will adapt.
What do physicians and patients think?
The distinction between detection and diagnosis is relevant when looking at physicians and public acceptance of autonomous imaging AI.
Radiologists are wary of relying on AI to diagnose cancer. In one study, medical professionals had to assess the accuracy of chest X-rays and diagnostic information, some of which was incorrect. Although part of the advice was identified as coming from an AI system, all of the advice was actually provided by human specialists. Yet radiologists rated the diagnosis as poorer when it appeared to come from an AI system.
The AI rule-out system we described above would not make a cancer diagnosis itself, only remove scans that don’t require action from the radiologist’s worklist. This would drastically reduce the workload, which is a good reason to believe that, should such a system be in place, physicians would be interested in using it.
Until then, radiologists urge caution. In a 2020 letter to the FDA, the American College of Radiology and the Radiology Society of North America asked the regulator to wait until assistive AI is widely adopted before approving autonomous AI.
There is a similar trend among patients. According to a survey, most patients were at ease with AI reading their chest X-rays, but much fewer said the same about AI diagnosing cancer. Most people would feel uneasy if an algorithm made decisions about their healthcare without any human input.
Education is crucial, another study shows. Patients don’t know when or how AI supports their doctors. Intriguingly, this study discovered that the public has much higher expectations for diagnostic AI than radiologists.
Are mistakes only human?
We may have raised quite a few questions on autonomous AI in medical imaging, but ultimately, it comes down to one: Do we accept a computer making mistakes just as humans do? No AI system is ever 100% accurate – there is always an outlier, an exceptional case that the AI will miss.
Yet, although computers are not perfect, they can be kept to a higher standard than humans. AI may be, and, in some cases, already is, more accurate than radiologists. And this is why it’s worth pursuing autonomous AI in medical imaging.
In this article, we outlined the need for an AI-based system to rule out normal CTs in lung cancer screening, the complexity of developing it, and the decisions facing the medical community, the regulators, and the public. Considering all the unknowns, we estimate that it will take five to ten years to have sustainable answers to the pending questions, the resources, and the acceptance needed to build the solution.
As Aidence, we are committed to taking on these challenges and expanding the possibilities of improving healthcare with AI.