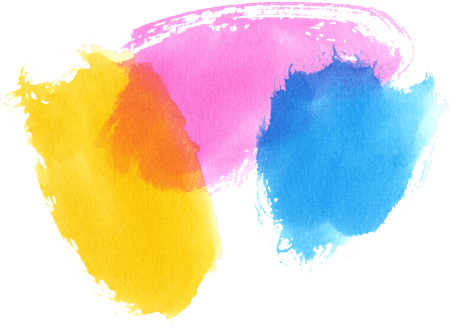
Starting this month, the UK’s National Health Service (NHS) requires all suppliers of new high-value contracts to publish a carbon reduction plan. The measure is part of the NHS’ net zero by 2030 ambition. It’s likely that other healthcare organisations will follow.
Sustainability was also high on the agenda at the European Congress of Radiology earlier this year. Sessions and panels dealt with the topic beyond medical imaging, looking at the suppliers supporting clinicians, such as manufacturers of medical equipment, services, and solutions, including artificial intelligence (AI).
The development of AI is known to be carbon-intense. As AI tools become an essential part of medical practice, healthcare providers adopting them will consider their environmental impact. But AI for clinical use is still in its early stages, with vendors prioritising the establishment of sustainable business models.
So, save the planet or do good business? We argue that you can do both. In this article, we look at the sources of AI’s carbon footprint, calculate our own emissions, and show that reducing them can be a win-win strategy.
The source of the matter
To understand why AI affects the environment, we need to visit data centres, hosting the millions of computers used to store, transfer, and access digital information. Data centres use 1-1.5% of global electricity, accounting for about 1% of global greenhouse gas emissions. Not far behind the airline industry, which is at 1.9%.
Their energy consumption is higher than some whole countries. They also take a sizable architectural footprint. Their scale – tens, even hundreds of thousands of square meters – means they utilise a large amount of resources – i.e., land and water – and generate waste.
For AI workloads, GPUs (Graphics Processing Units) are the main driver for this carbon intensity, because they require a lot of energy.
Yet, for a long time, the conversation around data centres and GPUs remained on technical or cost-efficiency points rather than ethics. Their environmental impact is less evident than for other industries. The constructions themselves are virtually invisible, sometimes, literally, by being built underground.
Recent developments have brought the carbon footprint to light. Training AI model ChatGPT-3 led to emissions of more than 550 tonnes of carbon dioxide equivalent (CO2e), comparable to a passenger taking 550 return flights between London and New York. These emissions are set to increase as the model becomes integrated into search engines, serving millions or even billions of users.
The AI solution
While AI has a significant carbon footprint, what does that mean for radiologists using AI?
We’ve calculated the carbon emissions of training and running our radiology AI solution Veye Lung Nodules.
First, some background information:
- Veye Lung Nodules automatically detects, measures, classifies, and tracks the growth of pulmonary nodules on chest CTs, then delivers its analysis into the radiology workflow.
- Its detection feature was trained with 45,000 chest CT scans from the American National Lung Screening Trial (NLST).
- Veye currently analyses thousands of scans each week across roughly 100 hospitals in ten European countries, including France, Germany, and the UK.

The calculation
As a disclaimer before we start, in reality, we haven’t emitted the below amounts of CO2e, but, in fact, netted zero. More details in the coming sections.
While all software gets built and run, with AI-based software we need to train a model which is data and energy intensive. It also – often, and in our case – requires specialist GPU hardware so that it is fast enough to run it.
Training the model
Our research team uses a private hardware stack for training and experimentation, hosted in the Netherlands. Most Dutch data centres are ‘greened’ and held to a strict standard of energy efficiency. Consequently, doing our modelling work in our home country is generally a good choice for the planet.
Veye’s machine learning model isn’t actually one model; to do the complex work of medical image processing requires a multi-stage approach to model architectures. So, we have included some approximations in our calculation.
We considered the number of experiments and their length, the duration of training and testing runs. We used this Machine Learning Emissions Calculator and The Netherlands’ 2022 grid efficiency stats to derive the carbon equivalence.
The estimate obtained was ~220 kilograms of CO2e for the final training of Veye Lung Nodules. It is roughly 0.04% of the 550 tonnes of CO2e for GPT-3.
This is not surprising since our models are relatively small. Training is possible on multi-GPU machines in weeks rather than thousands of GPUs over months. The environmental impact of training and validation is, therefore, of marginal concern.
Even so, our approach to model training has changed in the last year, for various reasons, like better GPUs, training pipelines, and new model architectures. We now estimate the same training phase is nearer to 25% of the above value over days, not weeks.
Running the model
In a previous article, we explained why we use the public cloud to run our services. Our figures originate from the data we can get directly from Google Cloud’s carbon footprint metrics.
We started from the total volume of scans processed in a given month across all the sites using our solution. Using an approach inspired by FinOps, we looked at the cost and carbon intensity per scan. These units together explain how much we’re spending as a business to process each chest CT scan and what our carbon emissions are as a result.
To put the results into context, we’ll compare them with the emissions of running a CT scanner. Research quoted in AuntMinnie indicated that one CT scan generates 3.6 kilograms of CO2e, and roughly 6,240 kilograms each month.
Our first finding: the emissions per scan for running our model on chest CTs can add ~3% to the emissions of each scan.
Our second finding: the total monthly emissions for our AI analysing and reporting on lung nodules for all our customers leads to roughly 95% of one CT scanner’s monthly output.
Life cycle
At this point, it is impossible to accurately estimate the total life cycle carbon footprint of Veye Lung Nodules. The data is insufficient, especially considering we are in a growth stage as a company.
That said, there are other areas a business can and should consider, such as office energy supply, business travel, and remote working. We’ll save these for a follow-up blog on our commitment to environmental sustainability in all aspects of the business.

The link with costs
Many think the most energy intense and costly part of developing AI-based applications is training the models. As an AI company deploying radiology solutions to hospitals across Europe, we know that the algorithm is just a fraction of the work.
Our algorithms don’t have billions of parameters, so they don’t require large numbers of GPUs and months of training and validation. The carbon emissions and costs of training also reflect this.
However, as more clinicians use our solutions, we need more computing resources. Our costs to operate this infrastructure would be driven in a similar way – as would our carbon footprint.
Today, the CO2e emissions from running our model represent ~55% of our overall footprint. This might grow more or less in line with the volume of work we do and our cloud spending.
Unless we do something about it.
Ways to reduce the footprint
What are we doing now and planning to do next to reduce our emissions?
Carbon neutral cloud provider
Because we’re using Google Cloud, our footprint is already carbon neutral. Google has been matching all its electricity consumption since 2017 and has committed to operating all its data centres on carbon-free energy by 2030. As long as there are GPUs, we try to select the cloud regions with the lowest carbon intensity, i.e. those that mostly or entirely use green energy.
Thus, we do not emit a scanner’s worth of CO2e every month; we effectively emit zero. The calculations above reflect our equivalent impact if we were on the grid.
Our research work runs in a private data centre. However, the supplier also sources energy from a certified green energy supplier, so the effect on real emissions is mitigated.
Nonetheless, there are secondary environmental impacts to data centre use that cannot be reasonably ignored, such as land and water use. So, despite the above, there are still good reasons to minimise our footprint.
Pay-as-you-go model
Infrastructure cost and carbon footprint are strongly linked by their energy usage. We’re already using a pay-as-you-go model for our computing resources. So, when we find ways to do the same amount of work with less infrastructure, both costs and footprint will decrease.
We have already changed the way we do our research, including model training. Furthermore, we are continuing to look at improvements that might reduce or even neutralise our footprint in those areas.
Adaptive IT architecture
We are taking major technical steps towards a more adaptive IT architecture. Our physical use of resources will follow the workload of radiology departments. For example, we require more GPUs during a busy morning than at night, and we see how different radiology departments work from one day to the next, or even between mornings and afternoons.
It isn’t very different from the idea of only running the heating when you know you’ll be home.
And it makes a huge difference.
Ethical growth
In over seven years of activity, it has become clear that our AI solutions are solving real-world clinical problems. We are, therefore, determined to expand our install base and portfolio. At the same time, our growing operation has a growing environmental impact, and we cannot, ethically, look away. Thus, our commitment to reducing, reusing, and recycling our resources.
Our calculations, though arguably imperfect, show a clear relationship between carbon emissions and business costs. Decreasing our carbon footprint as an AI company and growing in a sustainable way is also good for the bottom line. Without claiming to have found a one-size-fits-all solution for all companies in our sector, we encourage our industry to give our example a go, or at least start the discussion.