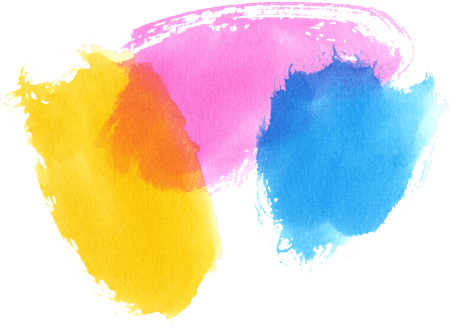
Covid-19 has brought profound changes to lung cancer care. In combination with staff shortages, it has led to the UK’s National Health Service (NHS) now facing a severe backlog. Seven in 10 NHS England trusts are missing the target for all cancer referrals, and the number of people waiting too long for treatment is almost 500% higher than a decade ago.
Efforts to address the delays are underway. More community diagnostic centres have opened. We’ve seen promising results from the Targeted Lung Health Checks, a soon-to-become nationwide lung cancer screening initiative. But more work must be done to catch up with lung cancer in the short and long term.
Imaging plays a fundamental role in cancer diagnosis and treatment and is often the starting point of the care pathway. So, when it comes to finding ways to beat the backlog, the radiology departments are a good place to start. Their workflow can improve by adopting emerging technologies, such as AI, to enhance image acquisition, prioritise cases, or support decision-making.
Radiologists who have been using AI for lung nodule management in targeted and opportunistic screening settings have noticed some of its benefits. Dr Katharine Johnson, Consultant Radiologist, Salisbury NHS Trust and Southampton University, summarised these:
“I strongly believe the accuracy, speed and reproducibility of our reports have increased significantly. We have reduced the number of unnecessary follow-up exams and have been able to highlight more quickly those that need intervention.”
In this article, I outline recent clinical research that supports the quote above and propose three ways radiologists can use AI to tackle the post-pandemic lung cancer backlog.
Go through the volume of scans faster
The Royal College of Radiologists census confirms it every year. There aren’t enough radiologists to review the increasing numbers of medical images. The current shortfall is at 29% and set to reach 39% by 2026, putting the staff under immense pressure.
With the help of AI, it may be possible for the existing workforce to report on the large volume of scans at a faster pace. AI-based lung nodule management has been shown to speed up CT reporting time in routine clinical practice. A study with two radiologists indicates that reporting pulmonary nodules using our AI solution Veye Lung Nodules reduces reading time by an average of ~ 40%. Veye helps achieve these time-savings by automatically detecting pulmonary nodules, measuring, classifying, and tracking their growth.
In the above study, the radiologists concluded each review with a patient management recommendation based on the British Thoracic Society (BTS) guidelines, like using volumetry in their follow-up decision. AI-based automated volumetric measurements, including growth percentage and volume doubling time from the most recent prior, contributed to the reading time reduction.
Automating simpler tasks and providing meaningful data-driven insights means radiologists can make better use of their time, attending to patients or focusing on complex cases. For patients, a radiologist getting back to them with their results faster means less time to wait for and worry about the outcome.
Maintain or increase accuracy
Early diagnosis is essential to better outcomes in lung cancer care, as survival is directly linked to the stage at which the cancer was found. Detection in the earliest stages also reduces treatment needs and costs, easing the weight on the healthcare system. Thus, as we aim to report on scans faster, we must also ensure we catch developing lung cancers accurately and on time. It applies to both in targeted screening and routine practice, by adequately following up on incidental nodules.
In both settings, AI is well-suited for the precise detection of elusive lung nodules on CTs. Real-world use has demonstrated high sensitivity for detecting nodules using AI. In a study at a large teaching hospital in the Netherlands, authors analysed the performance of our AI solution on 145 patient scans. They obtained a sensitivity of 88%, with a negative predictive value of 95%.
A timely diagnosis can be life-saving for lung cancer patients, and AI might just be the technology that can empower physicians to deliver it.
Streamline lung cancer screening programmes
In recent good news, the UK National Screening Committee recommended the rollout of a lung cancer screening programme across the four nations, mentioning the Targeted Lung Health Checks (TLHCs) programme in England as a fitting starting point. So far, the checks have caught 77% of the diagnosed cancers at stages one and two.
Expanding lung cancer screening will save or improve the quality of many people’s lives. However, it will also add a high additional volume of scans, which does not help with the backlog. So, how to make screening feasible in the current context? Dr Graham Robinson, President of the British Society of Thoracic Imaging, said:
“I can’t see a way that you should or could do lung cancer screening without AI.”
The majority of TLHC sites are using AI for lung nodule management and reporting. Radiologists rely on, for instance, AI-based quantifications, some of which are difficult for humans to perform, and for support complying with the reporting protocols. For more on their experience and our learnings from supporting multiple TLHC programmes, have a look at my previous article.
Moreover, AI-based automated volumetry provides an excellent opportunity to increase the impact of lung cancer screening programmes. Although many NHS trusts still use diameter measurements as the default, evidence supports the use of volumetry to assess pulmonary nodules. Dr Robinson, in fact, considers automated volumetry key to successful lung health checks.
Preliminary study results presented at this year’s European Congress of Radiology support this standpoint. Researchers concluded that AI-based volumetrics could avoid further follow-ups for one in five patients. This is significant, as early discharge reduces radiation exposure and anxiety. It also decreases hospital costs via fewer CT scans and appointments.
Thus, there are solid arguments to believe that expanding lung cancer screening programmes should go hand-in-hand with accelerated AI adoption.
Coming through
Are we set to face a lung cancer epidemic due to the backlogs and constraints in healthcare? We have reasons for concern but also strong hope for solutions that can help us prevent future harm.
Early lung cancer detection, both by targeted screening of at-risk populations and opportunistic screening in routine practice, is crucial to avoid a build-up of late diagnoses that would burden healthcare systems. In both clinical settings, studies into AI-based lung nodule management are encouraging.
We are also undertaking further research with the NHS and European partners to understand and highlight other opportunities to address some of the CT backlogs. Two ongoing projects are INPACT,looking into AI’s effect on radiologists’ decision-making, and PINPOINT, aiming to implement nodule clinics in hospitals and establish them as the standard of care. The latter is also a consideration in the Getting It Right the First Time (GIRFT) national speciality report for lung cancer.
Physicians need and deserve all the help they can get right now. In the words of Dr Johnson:
“I have significant anxiety at the thought of losing this [Veye Lung Nodules] and going back solely to the previous software, as I am sure we will not be able to maintain our current report turnaround.”