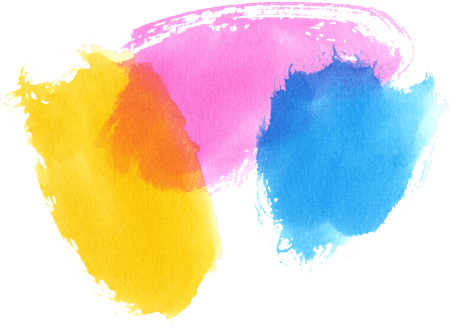
The yearly World Lung Cancer Day and Lung Cancer Awareness Month are opportunities to raise awareness about the leading cause of cancer deaths worldwide. Through open conversations, we can spread knowledge and understanding of the disease, from risk factors and prevalence to diagnosis, treatment and life expectancy.
As a former National Health Service (NHS) doctor now working in healthcare technology, here’s why I think artificial intelligence (AI) needs to be part of the conversation too.
Hype, myths, and truths
Admittedly, when I first worked in the NHS, I knew very little about artificial intelligence. If I heard AI mentioned in the context of healthcare, I pictured a robot with a stethoscope around its neck, probably because of media hype and rumours that human doctors would one day be redundant. Being fairly sure this was unlikely to happen in my lifetime, I tended to dismiss AI as irrelevant.
But when I began training to specialise as a radiology doctor (i.e. medical imaging), I became aware of technologies that were being developed to help detect and monitor disease through this medium. Today radiologists are required to know about machine learning techniques and AI development, because of the increasing use of AI software in the specialty.
My particular interest, early detection of lung cancer, is one area of clinical radiology which has the potential to be optimised by AI. Having worked on projects in my hospital to improve the early identification of lung cancer, which in turn improves survival rates, I naturally wanted to explore how AI could contribute to these pathways. One example is automated detection; computers have the capability of detecting very early disease that can be difficult for humans to see.
AI can therefore assist radiologists (rather than replace them) by highlighting these subtle abnormalities on the scan to draw attention to possible sites of disease.
Pattern recognition
Part of a radiologist’s job is pattern recognition. During training, I initially learned to recognise normal scans (note: there is a big range of ‘normal’). Knowing what normal looks like makes it possible to identify abnormal scans. By looking at thousands of scans, I developed confidence in spotting subtle abnormalities.
In a similar way, a computer (note: one that is specifically built for the purpose of AI, using artificial neural networks that work like human brains) can learn to recognise features by being shown thousands of images. So, like a human, the computer can differentiate between abnormal and normal by learning from experience. (A very basic description of artificial intelligence!)
Interpretation
A radiologist’s job doesn’t stop at recognising abnormal images; they use their medical knowledge of human anatomy, physiology, disease and epidemiology, as well as their understanding of medical imaging science (X-ray, magnetic resonance (MR), nuclear and ultrasound) to interpret abnormal findings. By gathering all the relevant information about each patient, they piece together the puzzle and determine the most likely diagnosis and appropriate management plan.
The complexity of gaining relevant clinical information to determine the most likely diagnosis is why our radiology doctors are not going to be replaced by doctors anytime soon.
Do we need AI in healthcare?
Yes. Why? Well, for a start, we don’t have enough radiologists.
Medical imaging plays a central role in diagnostics. As imaging techniques continue to evolve and we strive to detect the disease as early as possible, requests for scans continue to increase. Radiology departments have raised concerns that they cannot keep up with the demand for scans, and are worried about the impact on patient care. My previous blog discusses this in more depth.
Collaborative working
AI has the potential to assist overloaded radiology departments by performing tasks that are better suited for computers, such as measurements of tiny abnormalities in the lungs. Where humans vary in their accuracy, computers can provide greater precision and consistency.
Another advantage of computers is that they aren’t susceptible to stress or fatigue. Software outcomes remain consistent throughout a 24/7 week regardless of external pressures, whereas human levels of concentration wax and wane. Shift patterns, working twelve consecutive days, or 12-hour shifts without adequate breaks take a toll on our doctors… They are humans, after all.
By employing AI software to perform specific, repetitive tasks, computers can provide time savings and relieve some of the pressures on radiologists. AI can free up time for radiologists to explain results to patients or to perform practical procedures such as obtaining tissue samples. Patient and doctor interaction is one of the most rewarding parts of being a radiology doctor and a really important part of patients’ experiences during illness.
Will patients’ journeys change because of AI?
No. You will still see a radiographer when you come for your scan and see your doctor in order to discuss your results once your scan has been reported by a radiologist.
This diagram, for example, shows how Veye Lung Nodules, our AI software, is integrated into the diagnostic pathway:

Veye Lung Nodules helps radiologists to detect and measure small, subtle lung abnormalities on chest CT scans. It also analyses previous scans — if a patient has been for a scan before — and compares the current and previous findings. In this way, Veye Lung Nodules works as a radiology assistant, making the reporting process more efficient.
We developed Veye Lung Nodules by training our software on 45,000 scans. Note: there are patient privacy and data protection regulations in place to ensure that scans used for developing AI software are anonymous. Once Veye Lung Nodules had learned to recognise early cancer, we assessed its accuracy by comparing results with expert radiologists. we did this using a new set of previously unseen chest CT scans.
AI software must comply with quality assurance standards and regulations that have been put in place to ensure accuracy and patient safety. Following external assessment of our software against these standards, we gained approval for clinical use. Veye Lung Nodules is now being used in clinical practice across the UK and wider Europe.
The future of AI and lung cancer screening
Numerous trials around the world have shown that we can reduce the number of deaths from lung cancer by screening at-risk individuals using low-dose CT scanning. Scientists, medical leaders and patient advocacy groups are recommending that governments implement national lung cancer screening programmes across Europe. Efficacy, cost and capacity within healthcare systems play roles in the decision-making process. Without adequate funding and workforce, screening programmes will not be feasible.
Leaders in healthcare are recognising the need to use appropriate AI software to support the workforce and ensure screening programmes are successful and sustainable. Through collaborative approaches to screening programmes, we can achieve robust, sustainable pathways for early detection and continue to reduce the number of lung cancer deaths worldwide.