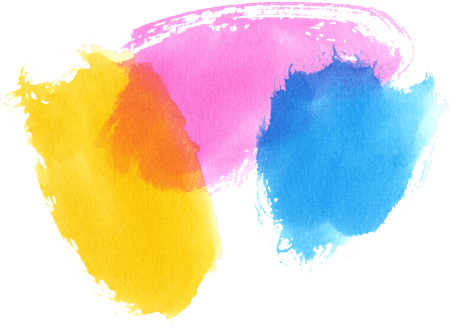
The inspiration for this article came from a tweet by Dr Amine Korchi, neuroradiologist and medical technology specialist:

As the co-founder of an artificial intelligence (AI) company, I fully agree. AI is a powerful technology with great potential to transform healthcare. It is also a hyped-up innovation that does not constitute the complete solution to the challenges radiologists face today.
Radiologists, and clinicians in general, need to analyse more and more data to provide diagnostic or treatment recommendations. AI tools support their analysis. But prior to the decision-making, they need to collect all the relevant patient data, often from different systems. Many are using notebooks, spreadsheets, files and folders, making the workflow time-consuming and error-prone.
We need more software than AI because there is an equal or even bigger need for aggregating complex patient data to empower medical specialists to interpret it, supported by AI. In addition, AI’s clinical usability relies on workflow integration, which is facilitated by software.
This article is my take on why radiology, and, one step further, oncology, need intelligent software products more than AI algorithms.
Software needs in the cancer pathway
Medical imaging is often the start of a diagnostic and treatment pathway, and oncology is no exception. Further down the cancer pathway, diagnostic and follow-up decisions rely on multiple clinical parameters for each patient, e.g. medical scans, tissue samples, and lab results. Most times, these parameters are stored in different systems.
Manually collecting data for patient management and interdisciplinary decision-making meetings is a time-consuming operational task. Automating it would be a productivity boost for clinicians.
To illustrate, let’s take a closer look at two stages in the lung cancer pathway. We are developing software products to address both use cases.
Patient management
An AI system can detect elusive abnormalities in the approximately 300 images in a chest CT scan without being impacted by the distractions or work pressure to which radiologists are exposed. But what happens after nodule detection?
The detection of one or several actionable nodules results in a report for follow-up actions. Depending on hospital protocols, follow-up may consist of making sure patients are invited for another scan after a certain period and checking that they have attended the appointment.
There is usually no system in place to streamline these steps. Clinicians and nurses enter the information and activities in a spreadsheet, making it difficult to track many cases over time.
A software tool could pull the report out of the radiology system, make an automated list of follow-up activities, and remind clinicians (and patients) of an upcoming or missed appointment. For a smooth patient management process, the software must connect with the PACS, RIS and potentially even the patient scheduling system. Solving this simple problem with integrated software would save a lot of time and effort.
Multidisciplinary meetings (MDTs)
In the scenario above, a follow-up scan showing nodule growth – thus, a potential cancer patient – is referred to a tumour board or multidisciplinary team meeting, MDT for short. In these meetings, different specialists – e.g. radiologists, pathologists, oncologists, lung specialists, and cancer nurses – discuss the case and decide how to treat the patient.
Clinicians struggle to find the time to collect all the relevant information about the case and interpret it in preparation for the meeting. If the patient cases are incomplete, it is also impossible to provide recommendations during the MDT, resulting in more time loss.
Software can collect and interpret the information needed during MDTs, and display it in one interface to support decision-making. This is a complex software engineering task because it requires integrating disparate systems, e.g. EMR, radiology, pathology, and laboratory systems.
Based on our current AI capabilities, we are more and more asked if we can provide treatment recommendations and prognostic information about potential treatment response. The question reflects the expectation that AI can perform this type of advanced prognostics. In reality, it is not the case yet. The first step is bringing all the data together, and only then can we potentially apply AI to that data.
Software engineering means ‘plumbing’ all the systems to bring data into the workflow. To continue with this analogy: data coming out of ‘pipes’ will need to be ‘cleaned’ to ease interpretation. All this data piping and cleaning isn’t very sexy; no wonder software is less glamorous than AI.
From algorithm to product
When I reached out to Amine Korchi to get some background on what triggered him to write the message, he made a strong, critical argument:
“The current landscape of companies are focusing on developing AI “Apps” or features. These features, if not integrated into a product/software, will not bring enough value to radiologists.
Without Health IT, there is no radiology and no AI. AI can’t bring its long-awaited value without being melted with Health IT. AI should transform Health IT first before even thinking of replacing radiologists.”
Developing an AI-based product requires a lot more than algorithm training. Most of our team’s work is software engineering, followed closely by documentation and testing. We further spend time on service and maintenance, information security, quality assurance, and post-market surveillance (hang tight, we’ll be publishing a very insightful article about this next!).
At Aidence, we talk about our AI-based pulmonary nodule solution: Veye Lung Nodules, the device using deep-learning algorithms to detect and analyse pulmonary nodules on chest CTs. Nonetheless, credit for Veye Lung Nodules’ clinical value is also due to the software architecture that makes it possible to use it within the radiology workflow. This includes:
- Veye Engine: an accessory to the medical device, integrated with the hospitals’ IT (PACS) systems. It enables the query and retrieval of CT studies and the anonymisation of patient data. In other words, it provides Veye Lung Nodules with the data to analyse, then returns the analysis to the PACS.
- Veye Bridge: a gateway between the engine and the device. It encrypts the connection between the hospital network and our devices, enabling cloud integration.

Moving the centre of attention
Software has a critical function but gets less notice than AI. Amine summed it up very well:
“AI gets more attention than software because it’s sexy, while HealthIT (RIS, PACS, EHR) isn’t. (…) Advances in computer vision have been fast and impressive during the last decade, but radiology isn’t “just” a visual discipline and pixels.“
It is time to redirect the industry’s attention to the impact software engineering can have on medical imaging and, beyond, on care and treatment pathways. There are simple problems that we can solve with good old-fashioned software engineering. Automating data collection, I have argued, can be the leap oncology needs towards an optimal workflow.
Despite early doomsday predictions, AI will not replace radiologists. For the foreseeable future, AI will remain an assistive technology, performing repetitive and tedious tasks. The complex reasoning and responsibility for diagnosis and treatment decisions are up to the healthcare professionals. And, for AI to support their decisions, we again need software solutions to enable integration into existing IT infrastructures.
Clinicians’ time is better spent interacting with patients rather than looking for records and filling out spreadsheets. On the other side of medical AI or software there are patients, and improving their outcome is what makes any technology relevant.