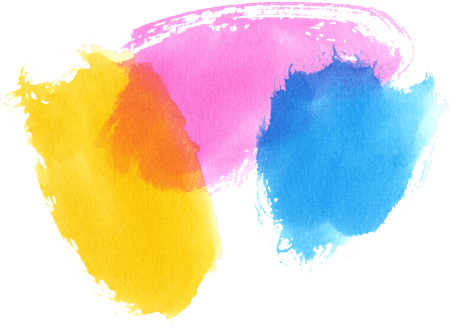
As a clinician, I was always interested in quality improvement. I enjoyed the logic of the clinical audit and quality improvement cycle and the satisfaction of measuring the positive impact of a successfully implemented change. A pull factor for me in MedTech was the opportunity to contribute to a far-reaching positive impact on healthcare systems (and people’s lives). I envisioned the same quality improvement cycle – but on a much bigger scale.
What I had not appreciated before joining a MedTech scale-up was the complexity of the quality management system. Even with the most rigorous development and validation of an AI medical device, it is not possible to, realistically, capture every clinical scenario pre-market. In my opinion, AI healthcare solutions are never “final” but are part of evolving imaging technologies, data, and clinical and regulatory guidelines. It is, thus, essential to correctly and continually monitor the safety and performance of the device, proactively and reactively.
In this article, I outline Aidence’s quality improvement process (QIP). To show that we practice what we preach (and encourage transparency in the industry), I first share a recent post-market incident that resulted in a Field Safety Notice (FSN) and the steps we took to solve it.
My premise is that a real-world example of an incident with an AI device will provide a concrete and relatable argument for the importance of rigorous post-market surveillance (PMS). It might also help clinicians and engineers better understand the potential risks of using AI in clinical settings and increase the healthtech industry’s commitment to quality.
A real-world incident
A hospital using our AI-based pulmonary nodule assistant reported a discrepancy in its analysis. Over the past months, we addressed the incident and followed the steps to avoid its repeating. This is an example of reactive (rather than proactive) quality improvement.
To provide context, I will outline how our AI solution works. Veye Lung Nodules is integrated into the radiology workflow, where it automatically analyses every eligible chest CT scan. On default settings, it detects 90% of all pulmonary nodules present on the image. It then returns their location, size, and type (solid or sub-solid) for the radiologist to review.
Veye Lung Nodules also retrieves the most recent prior study, if available, and calculates the volume growth percentage and volume doubling time per nodule. The nodule analysis report includes a 3D representation of each nodule.
One of the hospitals using Veye Lung Nodules noticed a difference between the outline of a nodule and the calculation of its volume growth. Looking at the nodule, they did not see a change from one scan to the other. Veye’s measurements, however, indicated an increase in volume. Based on clinical guidelines, volume growth warrants continued patient follow-up.
The image below shows how the device presents its results and which areas did not match (not the case in this example):

One of my responsibilities as Medical Director and Clinical Lead for Post-Market Surveillance (PMS) is to regularly review clinical feedback and determine the next steps, together with the ‘feedback team’. In this case, we found that Veye Lung Nodules was not performing as expected, which is very rare. We thus initiated the CAPA procedure (Corrective and Preventive Actions), which triggered the quality improvement cycle.
The root cause and possible impact
Our tech team used the Five Whys methodology to identify the root cause of the incident. They concluded that the difference originated from a technique to represent an image at different scales. Whilst the volume calculation output was affected, the other clinical features of Veye Lung Nodules – detection, classification, and diameter measurements – were not.
Our in-depth impact assessment indicated that the discrepancy could lead to a different clinical decision in up to 4% of all cases in which volume or volume growth is taken into account. To further pinpoint the risk, we considered the following:
- Radiologists take a holistic approach when determining the follow-up recommendation. Whilst they follow clinical guidelines, they will also consider each individual’s risk factors, such as smoking status, family history, or comorbidities.
- Veye Lung Nodules is used as a second or concurrent reader, which means a certified radiologist must check the results.
- Although the likelihood of an incorrect decision seems low, the possible patient harm of a wrong follow-up is serious. Worst-case scenarios would be an unnecessary invasive procedure, such as a biopsy, resulting in complications or a discharged early-stage lung cancer.
Our risk analysis matrix helped visualise and weigh the likelihood of an incorrect clinical decision and its impact on individual patients. To understand if any wrong clinical decisions resulted from volume discrepancy, we offered all hospitals a retrospective analysis of all studies processed by Veye Lung Nodules.
Corrective and preventive actions
1. Field Safety Notice
In line with our procedures, the first action we took was issuing a Field Safety Notice (FSN). The aim is to inform customers, authorities, and our notified body of the issue, following the medical device regulations (e.g., in the Netherlands and the UK).
We asked Veye Lung Nodules users to take two preventive measures: verify the volume and volume growth of the pulmonary nodule and inform other physicians of the issue. Based on the risk assessment, we decided that it was not necessary to stop using the device; its other features were working correctly.
Through clear communication, we could mitigate the risk of users relying on volumes without additional checks, whilst we fixed the underlying issue.
2. Product upgrade
Our data science team promptly fixed the discrepancy in the device, and our engineers released the upgraded version of Veye Lung Nodules to all hospitals within two weeks. From that moment onwards, radiologists could be confident that Veye’s volume calculations for a nodule match its visual segmentation.
3. Retrospective analysis
Two hospitals requested a retrospective analysis, adding up to approximately 4,500 chest CT scans. The analysis consisted of a comparison between the results provided in the previous and the new version of Veye Lung Nodules.
To minimise the workflow disruption for clinicians, our tech, medical, and regulatory specialists agreed with radiologists and the hospital IT team on a project plan.
The investigation into the actual clinical data demonstrated that about 1% of these scans were affected by the incident. Radiologists at the two hospitals reviewed the 1% of cases again and concluded that the volume difference had not resulted in any incorrect clinical decisions. Thus, no harm had come to patients, a very reassuring outcome.
4. Actionable improvements
To prevent future incidents, we reviewed our risk management, design and development procedures, and software requirements. This stage is an opportunity to challenge oneself: what can we change moving forward to deliver better products and define better processes? How can we better communicate as we grow as a team?
We identified three concrete improvements, implemented these, and are currently monitoring the impact.
The quality improvement cycle
Developing technology, it is tempting to focus on the next solution or a product feature and measure success on how much or how quickly we can deliver. However, we should look back, assess the impact of what we’ve implemented, or pause to question the way we’ve been doing things. Otherwise, how do we know there isn’t a better, more effective, and efficient way to serve clinicians and their patients?
So, as a first consideration, MedTech companies need to factor in time to look back and reflect as part of their quality improvement cycle. This is no different from healthcare practices.
The starting point for any quality improvement process is an audit through which we, as an organisation, ask ourselves: do we need to change? It can be the result of identifying an issue (reactive) or evaluating if we are achieving our goals and meeting customers’ expectations (proactive).
The second step is introducing change by looking at ways we could have done or could do things differently. It is questioning the status quo: Have we considered alternative ways?
Once one identifies an improvement or new standard, a change management process follows to get all stakeholders on board. This stage implies collecting and analysing facts and data to argue for the change.
When a change is implemented, we assess whether it has the intended impact by measuring this impact through a re-audit. If the result is not up to standard, we need to try something else; thus, the cycle starts again.
The clinical audit/quality improvement process is a cycle, as visualised below:

The quality management principles
Quality improvement is guided by seven overarching quality management principles, defined by the International Organization for Standardization (ISO). The relevant certification for medical devices in the EU is ISO13485, with which we comply.

Applying these principles to our products, processes, and organisation, we ask ourselves the following questions:
On a product level: Is our device still working the way it should?
- Are there new imaging technologies or acquisition/reconstruction parameters that could impact the performance of our device? (e.g. new CT scanners)
- Have clinical guidelines changed? (e.g. the British Thoracic Society (BTS) guidelines for lung nodule follow-up have been updated recently)
- Are there new use cases that require nuanced configurations to make sure the device is supporting best practices? (e.g. Fleischner guidelines use average diameters, whereas BTS guidelines use the longest diameter)
On a process level: How are we tracking or monitoring the performance of our device?
- Can monitoring be improved as we have more users/analyse more scans, therefore processing more data? (e.g. analysing trends in aggregated data, possibly against a benchmark)
- How will we assess the initial and ongoing impact of the change? (e.g. clinical audits)
On an organisational level: Are our processes suitable as we grow, or can we improve our procedures or communication?
- Does the structure of the organisation maximise engagement and efficiency?
- Are we enabling strong internal and external relationships?
Four takeaways
The basic ethical requirement “First, do no harm!” translates in PMS terminology as ‘When in doubt, raise a CAPA!’. It is a guiding principle we applied to this incident and a confirmation of what I learned in my BSI training. It also continues to be my way of working moving forward.
Ensuring no patient harm requires risk mitigations, safety measures, and continuous assessment of the safety and performance of our device throughout its lifecycle, based on factual evidence. This is in line with one of the key ethical principles from the recently released WHO Guidance on AI for Health – promote AI that is responsive and sustainable:
“Responsiveness requires that designers, developers and users continuously, systematically and transparently examine an AI technology to determine whether it is responding adequately, appropriately and according to communicated expectations and requirements in the context in which it is used.”
Thirdly, I further realised the importance of strong working relationships between medical and tech teams. It is essential for someone with a clinical background like myself to work closely with the tech team. In my role, I can use my experience working in a hospital to help my colleagues’ doing complex technical work connect with patients and understand the implications of their work.
Finally, clear communication is a key component of the culture of any tech company which bears the great responsibility of serving healthcare. A tool like SBAR (Situation – Background – Assessment – Recommendation) might improve overall communication and feedback between technologists and healthcare professionals. It comes down to an enabling company culture in which addressing quality issues is a critical success factor.
A plea for transparency
A recent AuntMinnie article recognised the value of post-market monitoring by describing the changes in AI imaging devices over time as a ‘drift’. Dr Erikson’s advice:
“Have some method of monitoring that ‘drift’ and assessing performance changes when software upgrades occur.”
And when drifts occur, I would like to encourage the MedTech industry to be transparent and show how they worked to address them. It is how we can gain trust in AI medical devices and the organisations behind them, both from a clinician and a patient perspective.
Sure, we will all be worried that issues with our devices will damage our credibility. But being open and clear when handling an issue will strengthen relationships with healthcare providers and ultimately contribute to better patient care.