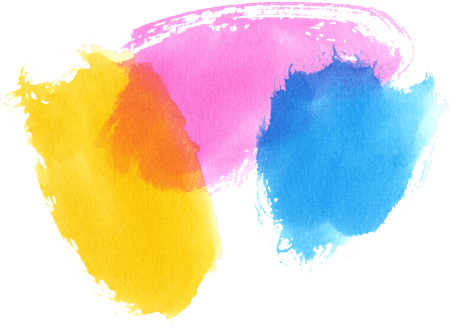
Medical device manufacturers often consider post-market surveillance (PMS) as an activity conducted by their regulatory affairs teams. At Aidence, PMS is a close collaboration between the Medical and Quality Assurance/Regulatory Affairs (QA/RA) teams.
The QA/RA team sets out the regulatory needs and maintains accurate overviews and documentation in the quality system. The medical team takes the responsibility of collecting proactive post-market data and planning the post-market clinical follow-up activities. This article combines the views of both teams.
Needs and drivers of proactive PMS
Overall, obtaining and documenting information from the market (reactive PMS, through complaints and user feedback) is relatively straightforward. Acquiring post-market data actively (proactive PMS) might, however, be more challenging.
AI devices have unique attributes that require more proactive PMS than other software medical devices (SaMD) or hardware systems. This is due to the following three reasons:
- There is little user experience with AI in healthcare since it is a relatively new technology;
- AI models are sensitive to bias, which is difficult to fully capture in pre-market stages;
- Changes in technology (e.g. CT scanners) or disease prevalence (e.g. covid-19) might cause an AI solution to drop in performance.
Manufacturers need to consider these characteristics to ensure the continued suitability of their products during clinical use.
With this increased need for proactive monitoring of the performance of software and AI devices, two strong drivers are leading us into the ‘golden age’ of PMS:
- SaMDs are increasingly cloud-hosted;
- Software within the healthcare sector increasingly allows interactions with users.
The above developments will allow manufacturers to move to live performance monitoring. Previously, manufacturers had to either rely on their users’ reporting or initiate their own post-market clinical follow-up studies (PMCF) to collect data.
Whilst this performance monitoring will not cover the full scope of PMS (e.g. it will miss user experience aspects), it will provide direct insight into product usage and device performance.
The benefits of post-market monitoring
The benefits of real-time monitoring of the use of an AI tool go way beyond the need to meet the stringent PMS requirements set out by the EU Medical Device Regulation (MDR 2017/745). We will outline the gains from the perspective of our work as Aidence.
Get a better picture of AI robustness
Veye Lung Nodules is our AI solution for pulmonary nodule management. It detects a percentage of all pulmonary nodules present on a CT scan, referred to as sensitivity. It may incorrectly classify healthy tissue as pulmonary nodules, referred to as false positives. We carefully analysed the trade-off between false positives and sensitivity (detection accuracy) before regulatory approval.
However, the pre-market setting presents limitations. Manufacturers can only try to get as close as possible to the actual accuracy of the device in clinical practice. This actual performance, nevertheless, may not align with the pre-market data.
AI medical imaging tools are validated on datasets that include a variety of settings, technologies, patients, etc., to ensure good generalisability. In clinical practice, however, the solution might be used on a specific population, specific scanning protocols, and, therefore, specific settings. As a result, it might not perform in line with its performance claims.
The FDA sets out (more) stringent clinical requirements than the EU for our type of software to enter their market (requiring reader studies in addition to stand-alone performance evaluation). Yet, it does not (currently) lay down strong PMS requirements for SaMD or AI devices. So, even though a device might be proven to significantly improve a radiologist’s reporting in a pre-market stage, its performance in clinical practice may not live up to the evidence, or even change over time.
Hence our plea for added emphasis within regulations on heightened PMS as compared to pre-market data. Post-market data provides a much better picture of the robustness of an AI device in clinical practice than pre-market data. We invite regulators and standard organisations to develop guidance for manufacturers of AI devices around their PMS expectations.
Mitigate safety risks
It is impossible to include all clinical scenarios and configurations in the pre-market clinical validation data of an AI tool. Hospitals use different protocols, scanner technology keeps advancing, and exceptional events or incidents occur. Active post-market monitoring ensures that new scenarios do not lead to unexpected results.
Recently, a hospital researched the impact of image reconstruction settings on the performance of Veye Lung Nodules. They found the device to perform as expected for the majority of common settings. Still, they advised caution when using multiple CT scanners and protocols, as there are differences in performance for less typical kernels (i.e. a reconstruction technique that sharpens the medical image).
Following this example, actively getting the data regarding a new scanner configuration at a hospital serves two goals:
- In the short term, the vendor and the radiology department can discuss the impact of the setting on the AI results. Additional user training should be considered as an option to mitigate the risk of continued use.
- In the long term, ensuring the AI application applies to the new protocol and poses no safety risks.
Gather trends for quality assurance
The use of our reporting solution, Veye Reporting, is a good illustration of the value of detecting trends. Veye Reporting extracts the results from our lung nodule application (from the radiology IT system) and automatically pre-populates an online screening template, saving valuable time in drafting the required report. If the radiologists agree to the findings, no additional actions are needed. If they disagree, they can adjust the results (e.g. add missed pulmonary nodules or remove false positives).
In the background, the software will soon start gathering aggregated data, e.g. the total number of missed pulmonary nodules and removed false positives by the radiologists. These figures will allow us to start monitoring, in real-time, the level of agreement of a radiologist with the AI findings and to compare data between clinical sites, patient populations and scanners.
Monitoring the radiologists’ agreement over time will start providing us with insights into trends and changes. For example, if we detect a sudden dip in performance, this can indicate changes in disease prevalence, technology or new staff in need of additional training. It may flag the need for software adjustments, i.e. by iterating on the learning of the model.
Save clinicians’ time
Research into the impact of AI solutions currently relies on clinical studies and audits. Both methods present advantages and disadvantages.
Clinical studies are a reliable methodology for deriving statistically significant results from large population sizes. We are currently designing studies to determine the wider clinical impact of Veye Lung Nodules as part of our AI award work. These studies are complex, time-consuming and expensive. Smaller district hospitals, in particular, have little capacity for conducting this type of research project.
On a smaller scale, clinical audits are good practice for capturing pragmatic and regular snapshots of real-world use. In the UK, one of the lung health check sites using Veye Lung Nodules performed an audit of the device as part of their quality assurance. In general, the Royal College of Radiologists (RCR) provides systematic and logical templates for NHS trusts to check compliance and performance against standards.
Audits are also a way for radiologists to steer AI developments. Their active engagement is needed to improve the adoption of new technologies.
Nonetheless, audits have less statistical power than studies as they use smaller samples. Moreover, they require physicians’ time for data collection and analysis.
Real-time monitoring of AI medical solutions could counteract some of the disadvantages of clinical studies and audits. Monitoring enables fast response and intervention without manual input from any clinician. Time-saving is a relevant benefit to consider with the ongoing workforce shortage and reports of burnout.
Post-market monitoring could further automate AI clinical audits. The device manufacturer would provide data to the hospital for quality management while also learning how their solution performs. A perceived disadvantage might be that the monitoring is led by the AI manufacturer, which, as a commercial party, may not enjoy complete trust. Therefore, the post-market surveillance system should be transparent to the users by, for example, sharing these insights with them in real time.
How to enable live AI monitoring
Post-market AI monitoring can be a game-changer in our industry, but it is no easy feat. Firstly, the AI software must be able to:
- Track the number of processed scans;
- Register whether a user agrees or disagrees with the results generated;
- Allow users to alter findings generated by the software.
Ideally, the last two steps are integrated into the clinical workflow. Integration is essential for an AI tool to augment the physician with as little disruption as possible (a point recently reiterated by the UK Royal College of Radiologists).
Unfortunately, as an AI vendor integrating into the Picture Archiving and Communication System (PACS), we are often limited by the PACS functionalities. Thus, as a second requirement, the IT infrastructure at the hospital must support user interaction.
From a regulatory perspective, as long as the monitoring is limited to the analysis of aggregated data (thus not including identifiable personal data), the monitoring activities are fully in line with the General Data Protection Regulation (or GDPR 2016/679).
A question of time
Live performance monitoring of AI clinical applications is a tangible goal, with enormous benefits for healthcare practitioners, patients, and the healthtech industry. The impact on PMS activities would set the bar for medical device quality and patient safety.
To realise the potential of this monitoring, we argue for two developments:
- An added regulatory emphasis on PMS; and
- PACS functionalities that enable data aggregation and analysis.
These developments would indeed aid in ushering in a new era in PMS for AI.