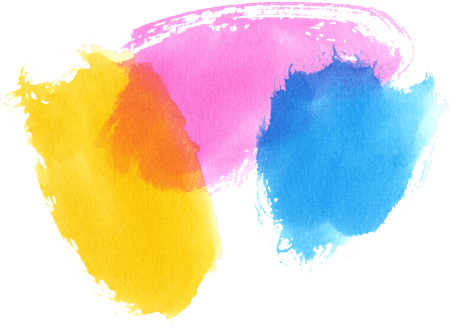
A speaker I find most inspiring is Liz O’Riordan. She is a breast surgeon who dealt with breast cancer herself and used her voice to empower patients and drive healthcare improvements. There is a lot to learn from her experience, and from breast cancer care and early detection.
Breast screening started over 30 years ago to try to limit the impact of the most commonly diagnosed cancer for women worldwide. Following positive results in trials, e.g. in Sweden or the Netherlands in the 70s, the EU proposed pilot programmes to members in 1986. Two years later, the UK began inviting women ages 50 to 70 for mammograms every three years, and more countries followed.
Both breast and lung cancer are major causes of cancer deaths in women, while lung cancer is the biggest cancer killer. Like we perform mammograms to detect breast cancer at an early stage, it is time to screen for lung cancer with CT scans.
To an extent, these efforts are already underway. In a previous article, I reviewed the benefits and developments of lung cancer screening initiatives. Today, on International Women’s Day, I propose we look at lessons learned from more than three decades of breast screening. These insights follow numerous conversations I had with experts in the field.
Single versus double reading
The European Commission guidelines on breast cancer screening advise that all mammograms are double-read (i.e. independent reads by two certified radiologists).
Nico Karssemeijer, the co-founder of ScreenPoint Medical and leading scientist in breast cancer imaging, explained that the initial argument for double reading was to avoid unnecessary recalls by increasing specificity. The screening of healthy women was met with resistance at first. Therefore, the negative effects had to be minimal.
The second read also improves sensitivity (by 5-15%, according to the guidelines), thus the chances of finding the more subtle, elusive abnormalities on the medical image.
The first learning from breast screening could be: screening is most effective when each medical image is double-checked.
With the introduction of lung cancer screening, the default is a single read. The main reason is, in my view, the lack of resources (in one of my first blogs, I discussed workload in radiology, drawing from my own experience). Additionally, whilst the mammogram solely shows the breast, chest CTs present a broader area with possible findings. A radiologist reporting on chest CTs will also look at the heart, bones, etc., and report on incidental findings. Logistically, it is a different, more complex workflow.
Another reason for the single read might be the limited cost-effectiveness of two consultants reading every scan, as some studies (here is one example) indicate.
Nonetheless, UK NHS trusts have been encouraged to set up ‘lung nodule multidisciplinary teams (MDTs)’, so the radiologist can refer a patient for review by a small team (rather than referring to the lung cancer MDT). This basically equates to requesting a second read and signals that the need for this additional scan review is present.
Quality assurance
The success of any screening programme depends on the benefit to patients outweighing the potential harms. A big concern is bringing back patients for follow-up scans which turn out unnecessary. These additional scans increase radiation exposure and create anxiety for the patient.
To monitor the value of the breast screening programme, the UK healthcare system uses a quality assurance framework. The process uses an online system to collect the individual reporting figures of each mammographer. Comparing them against the national standard provides an indicator of performance, with an above-average recall rate meaning a need for additional training or support. The evaluation also applies at a site level, regionally, and locally.
Nico Karssemeijer further emphasizes the importance of quality audits. Checking prior mammograms for possible errors allows for effective quality assurance on the whole process, especially radiologists reads.
Quality assurance goes hand in hand with adequate training. PERFORMS, a self-assessment and training platform for UK mammogram readers, is an interesting initiative. Participants get a set of real-world, anonymised, difficult cases to read and receive feedback on their interpretation.
What can we learn from these approaches, and what should we bring into lung cancer screening? Our second takeaway: the need for a system to provide training and monitor quality. I am confident that having a quality assurance system would help close the gap between healthcare systems. From my experience working within the NHS, I am aware of existing variations in early diagnoses and outcomes across the trusts. I suspect things are not much different in other countries. Ultimately, patients should (ideally) receive the same high-quality care regardless of where they are helped.
The role of innovation
A double read and quality assurance are both part of the ideal scenario for lung cancer screening. However, we must avoid straining the radiology workforce and adding a financial burden to healthcare systems. Innovation seems indispensable if lung screening is to become an effective and sustainable initiative.
Artificial intelligence (AI) applications for lung nodule management and reporting can deliver on the two takeaways from breast screening. We are witnessing the potential of technology first-hand, through our work supporting radiologists reporting on chest CTs as part of the NHSE Targeted Lung Health Check (TLHC) pilot.
The second pair of eyes
Our AI solution Veye Lung Nodules is currently used as a second or concurrent reader in several TLHC sites. It detects, measures, and tracks the growth of pulmonary nodules, then delivers the results back into the radiology workflow. Dr James Shambrook, Consultant Cardiothoracic Radiologist at University Hospital Southampton, compared using Veye Lung Nodules to:
“Having a high-quality trainee review each and every scan before you.”
This is just one sample of anecdotal evidence indicating that our AI application is that extra pair of eyes that may fulfil the need to double read screening scans.
Is an AI second reader consistently reliable and more cost-effective than a second radiologist? We honestly don’t know yet, because the real-world evidence is pending. As part of our recently won AI award, we will deploy Veye Lung Nodules in more hospitals and screening sites and conduct studies of its clinical and economic impact. Once completed, we will have a case for an AI-supported double reading.
Data aggregation and analysis
Artificial intelligence is great at integrating large amounts of complex data and highlighting patterns and trends. For a practical example, I’ll refer to our interactive reporting tool. Veye Reporting produces standardised reports of the findings delivered by Veye Lung Nodules.
The reports aim to support lung health checks and thus follow the pilot protocols and requirements. If we analysed these reports across different trusts and regions, we could define a benchmark for recall rates and highlight areas where this rate is too high. These results would generate new insights for quality assurance or improvement.
The image below shows how we envision this AI-based dashboard for monitoring quality in a lung screening setting. It uses mock-up data but follows our experience supporting lung health checks in the UK. The analysis provides the recall rates at a specific site and compares them against the programme target (eg. set by the NHS England) and the national average. It also looks at the results of the AI tool itself, measuring disagreement on segmentation, false positives, and false negatives.

AI quality monitoring
In 2018, the NHS screening programme reported a ‘computer algorithm failure’ that caused 450,000 women aged 68 to 71 not to be invited for a final routine breast cancer screening. The causes and impact of the issue were the topics of a detailed independent review. The conclusion was that a new algorithm from a screening trial was not compatible with the running IT systems, and the implementation process did not include alignment and checks.
Following many hospital deployments, we understand the complexity of technical implementation and the value of post-market surveillance. Integrating new software in a screening programme requires thorough quality monitoring of both performance and clinical use.
More work to do
There are many more nuances we could look into when designing a screening programme for any form of cancer. Some aspects will overlap between different programmes and may not need to be reinvented.
One area to carefully consider, Nico reminded us, is overdiagnosis. Before breast screening started, the precursors of breast cancers were largely unknown. As these programmes were rolled out, we learned more about the different types of cancer and their progression through stages. Thus, we know some cancers would not become symptomatic in a patient’s lifetime and would not contribute to death. Many argue these cancers are better left undetected. However, it’s often hard to tell the type of cancer from a scan.
The more work we do as Aidence, the clearer I see the value of technological innovations such as artificial intelligence to drive healthcare improvements we humans could not achieve alone.