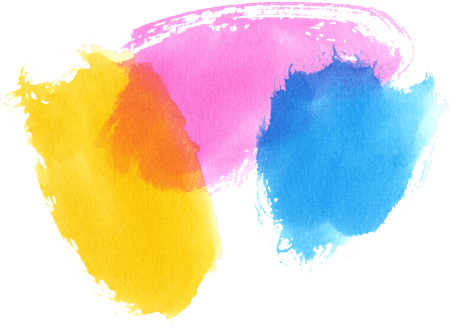
There have been many claims about technologies making healthcare better or more affordable. Artificial intelligence (AI) is one of the few innovations that can deliver on both these promises.
In this series of articles, I look at how AI can improve healthcare systems, without compromising on quality and costs. First, I review the impact of currently available AI applications. Then, I outline the most promising use cases for the near future. The series ends with ways to build a business case for adopting AI.
This first post is my high-level analysis of the impact of medical imaging AI today.
The 4P’s
To assess the impact of AI applications, I use what we at Aidence call ‘The P’s’. This is an internal framework that reflects the way our solutions support improvements in cancer care.
The starting point for developing AI is a clinical need expressed by the healthcare practitioner, whose workload we aim to ease. The high accuracy of AI should support precision diagnostics and increase productivity across the oncology pathway. It also needs to prove a return on investment and be part of a cost-effective healthcare system, which correlates to pricing. Well-performing and integrated AI solutions contribute to better patient outcomes.
This overview is not exhaustive, nor are the factors exclusive. The framework forms our angle in the industry – and we are open to talk about it.
The pricing value driver will be the topic of a distinct blog post on the business case of AI solutions. Benefits for the oncology pathway is where I see AI heading, and I will also address this separately.
The current use cases for AI
AI currently plays a role in two areas of the radiology department: technical and clinical. The first two use cases below are part of the technical improvements that AI innovations bring. The last two are clinical applications.
1. Image acquisition enhancement
The first level where AI currently has an impact is on the device level, by supporting image enhancement. AI solutions can increase the clarity or sharpness of scans and reduce the time for their acquisition, in some cases from 45 to 30 minutes.
The benefits of these AI tools are clear. A sharper image is easier to read for the practitioner, and the precision of their reading is increased. The higher speed of acquiring a scan impacts productivity and increases staffing and scanner utilisation. Image acquisition optimisation with AI also has a positive impact on the patient, who spends less time in the scanner and receives a lower dose of radiation.
2. Workflow optimisation
AI-enabled systems are available to drive worklist prioritisation – placing potentially urgent scans to the top or flagging them; and allocation – assigning studies to the most appropriate and available physician. The latter is common in teleradiology practices.
Overall, I see limited value in workflow optimisation AI for medical imaging. The clinical suspicion raised by a physician already defines prioritisation and allocation. This suspicion, based on the patient’s history, symptoms, and examination, is key, and cannot currently be done without human input. I acknowledge that workflow management varies from country to country, and base my insights on experience from Western Europe and the UK. Nonetheless, reports from the US also indicate a minimal role for these tools.
Let’s consider an example. A patient arriving in the emergency room with shortness of breath will be assisted by a physician on duty. If the clinical suspicion is pulmonary embolism (PE), the physician will immediately involve a radiologist. Before the radiologist reads the scan, there is already a human-raised flag in place and the need to report straight away. This leaves little room for AI to optimise the process for practitioners, or increase their productivity.
For a backlog of non-urgent scans, a flagging system is relevant, yet only in combination with the detection of incidental findings. For instance, a lung cancer patient undergoing chemotherapy will undertake follow-up scans to check for remission. This patient is also at a higher risk for PE. In the absence of symptoms, their scan arrives in the backlog. With an AI system flagging potential PE, there is value in speeding up the treatment. However, this is not possible without a tool detecting the incidental finding in the first place. Pure worklist prioritisation AI usually does not show the location of the threat, as it is done on scan level and not slice level. The precision is hence limited.
Furthermore, not having a prioritisation tool in the scenario above is not life-threatening. The chance of detecting a serious incidental finding in a list of non-urgent scans is very small. Thus, it is difficult to justify the cost of employing this technology. The potential benefit should also be weighed against the risk of deprioritising a scan that has an important finding, but which the AI system has missed. The impact of having an AI optimisation tool is, therefore (most likely) positive for the patient yet in exceptional cases.
3. Detection of incidental findings
This level concerns the role of AI in analysing a particular incidental finding within the scan. Rather than attempting to assess the whole scan and prioritise it in the worklist, the AI would provide information about an abnormality that is not causing symptoms and is not the reason for the scan, yet it is important to identify and treat. An AI solution could, for example, detect coronary artery calcification (CAC) on chest CTs taken as part of a lung cancer screening programme.
Some radiologists are very good at looking for incidentals, while others overlook them. For practitioners, having an AI solution as a second eye looking over their shoulder gives them the confidence that they will not miss anything.
This increase in detection capability allows the radiologist to read the scan faster, which means higher productivity. A physician looking for subtle abnormalities on a chest CT does not have to read the scan using different view settings if the AI system automatically detects and highlights present nodules. Instead, they can use the time to focus on the reason for the scan and on answering the clinical question behind it. A time-saving benefit could greatly aid the radiology workforce facing an increasing workload.
Detection AI can also increase precision, although arguably to a lesser extent than quantification AI, which offers automated, detailed measurements.
It is undoubtedly better for patients to have AI spot incidental findings on their scans, as this prevents missing a disease that wasn’t on the radar. It is also an opportunity to intervene at an earlier, more treatable stage, before symptoms arise.
The challenge, however, is to build a business case for the incidental finding. Are costs saved, and, if so, for whom?
4. Quantification of findings
A recent editorial reconfirmed that many radiologists would welcome the support of AI algorithms with the repetitive, time-consuming reporting tasks. For the practitioner, automating, for instance, the quantification of lung nodules on chest CTs, including measurement and characterization, frees up time for more rewarding tasks.
By augmenting the radiologist, quantification AI can speed up decision-making, therefore increasing productivity. It can impact precision, particularly by providing volume or density measurements. These are measurements humans cannot perform, whilst their importance as clinical parameters is growing. For example, in a case reported by a user of our solution Veye Lung Nodules, consecutive chest CTs containing a lung nodule showed no visible change in nodule diameter. Yet the AI algorithm correctly returned a significant volume growth, triggering the intervention of the medical team.
For patients, adopting quantification AI solutions can lead to better, more personalised treatments. And, in cases such as the one I’ve just mentioned, it can be life-saving.
Limitations of current research
If looking to invest in medical imaging AI today, detection and quantification capabilities can drive the most value. This is supported by clinical relevance, user feedback, and anecdotal evidence. Nonetheless, whilst legitimate AI vendors have performed studies to show their AI’s performance, efficiency gain studies are still pending.
Kicky van Leeuwen, AI in Healthcare Researcher at Radboud UMC in the Netherlands, was involved in the creation of the AI for Radiology guide to solutions commercially available in Europe. She shares her view and poses several questions:
“We reviewed the scientific evidence of 100 AI products in radiology and only about a third even have scientific evidence. From those papers, most demonstrate the ability to detect or quantify something with a certain performance, but little studies have been done that actually show the impact of that. How does that change the judgement of the radiologist? How does that influence the patient’s treatment or outcome? Do we gain life quality or save money?“
At Aidence, we are working with partner hospitals to gather data-driven evidence and provide a clear overview of the impact on practitioners, patients and costs. We’ve made an important announcement about that recently.
The scorecard
The table below summarises this analysis of the impact of AI clinical applications on the four healthcare value drivers. As workflow prioritisation and allocation are anyway done by humans, AI adds little value there. AI solutions for the detection of incidental findings and for quantification, on the other hand, can make a difference in medical imaging today. For practitioners, time-saving systems are needed to deal with the high workload,
AI for the detection of incidental findings can also be a part of preventative medicine to improve public health. Patients diagnosed at more severe stages of their diseases are harder to treat, as treatments are less effective at late stages. These treatments are also more expensive for healthcare systems. Quantification AI can further play a role in precision diagnostics, enabling appropriate clinical decision-making. Again, this would benefit patient outcomes and remain cost-effective.

What will AI bring next? The next logical step would be diagnostics – combining the output of AI with current guidelines to enable the AI solution to return the diagnosis outcome. Beyond that, AI can play a role in prognostics. More on that in a future article.